RH genotyping by next-generation sequencing
Introduction
Next-generation sequencing (NGS) refers to a spectrum of nucleic acid sequencing platforms that succeeded Sanger chemistry and that share two salient features: a high-throughput and a massively parallel nature (1). With varying underlying chemistries, workflows, precision rates, read lengths, and instrument footprint, these technologies have evolved swiftly over the past two decades, allowing clinicians and scientists to sequence an entire human genome faster and at a steadily decreasing cost (1-3). NGS has thus become a foundational pillar of precision medicine (4,5). Its clinical applications have rapidly expanded and transformed multiple health care fields, including oncology, pharmacology, microbiology, and hematology. For transplantation, NGS is the gold standard for high-resolution human leukocyte antigen (HLA) typing in histocompatibility determination (6), and its potential role in transfusion for blood antigen typing and blood group discovery has been discussed widely in the literature (7-11). The Rh blood group, with its closely similar paralog genes and high degree of polymorphism, including complex structural variation and gene conversion events (12), represents a unique and impactful target for clinical genomics. We present here a review of the recent literature, with a specific focus on the application of NGS for donor and patient Rh blood group antigen typing.
Diversity in the RH genomic locus: NGS perspective
The clinical significance of Rh blood group alloimmunization and the extensive and complex variation of the RHD and RHCE genes have been reviewed in detail. The reader is referred to comprehensive literature available on the topic (12-15). This review will focus on the unique characteristics of this blood group locus as they relate specifically to NGS applications.
Amongst the 45 blood groups recognized by the International Society of Blood Transfusion at present (16,17), the Rh blood group boasts the largest total number of documented antigens, as well as the largest number of known genomic alleles. Table 1 lists currently available online blood group allele databases that include the RH locus. These electronic databases were created or updated more recently than printed book versions of this data (15,23), and they not only represent a valuable clinical and academic resource, but also a critical tool to enable NGS blood group interpretation pipelines.
Table 1
Author, publication, year | Database | Website |
---|---|---|
ISBT (16) | ISBT Allele Tables | https://www.isbtweb.org/isbt-working-parties/rcibgt/blood-group-allele-tables.html |
Wagner et al., Transfus Med Hemother, 2014 (18) | The Human RhesusBase | https://www.rhesusbase.info |
New York Blood Center Genomics Laboratory | RHCE Table | https://www.bloodgroupgenomics.org/rhce/rhce-table |
Lane et al., Lancet Haematol, 2018 (19) | Bloodantigens | https://bloodantigens.com |
Rophina et al., Transfus Med, 2022 (20) | Bgvar | https://clingen.igib.res.in/bgvar |
Möller et al., Blood Adv, 2016 (21) | Erythrogene | https://www.erythrogene.com |
Floch et al., Trans Med Rev, 2021 (22) | Rheference | https://rheference.org |
The table is limited to online resources publicly available at the time of this writing. ISBT, International Society of Blood Transfusion.
Known RH alleles are widely diverse: they may have one to multiple single nucleotide variants (SNVs), in addition to copy number changes, exon translocations from their paralog counterpart, and microconversions that lead to multiple hybrid versions of these genes (12-14). Intronic variants have not been extensively documented, with the exception of a few studies (24-27). Notably, RH SNVs listed in databases may actually represent the reference allele for the paralog gene. For example, the exon 1 RHCE c.48G>C variant, which defines the common RHCE*01.01 allele [reported with a frequency of 53.4% in the worldwide 1000 Genomes dataset by Erythrogene (21)], corresponds to the reference nucleotide call for RHD exon 1. Conversely, multiple RHD alleles carry two SNVs on exon 6, c.916G>A c.932A>G, which correspond to the reference exon 6 read for the RHCE gene, and could therefore be described as an RHCE exon 6 conversion. This diversity poses unique opportunities and challenges for NGS technology.
Detection of novel variants
The number of RHD and RHCE blood group alleles is not only vast, but also continuously growing—the RH locus is effectively a fertile ground for ongoing genomic discovery. The unbiased nature of sequencing platforms—as opposed to targeted molecular assays that test for presence or absence of known variants—provides an advantage for identification of novel alleles in this context. Table 2 provides an overview of NGS blood typing studies in the literature that have incorporated the RH locus; 17 of these report novel variants in Rh or in other blood groups (21,24,26,27,31,33,34,36-38,41,42,44,45,47,48,50). In addition, multiple novel variants are described in conference abstracts, although these are not easily searchable in scholarly databases and have not been extensively peer-reviewed.
Table 2
Author, publication, year | NGS targets | NGS total sample size | Sample details/data source | Ethnoracial diversity | NGS chemistry | Read length | NGS library preparation | Analytic approaches |
---|---|---|---|---|---|---|---|---|
Stabentheiner et al., Vox Sang, 2011 (28) | RHD | 26 | Blood donor samples with weak or uncertain serologic D typing | NA | Pyrosequencing | Analysis with reads >100, mean read length not specified | Targeted: PCR-amplification of RHD exons | Amplicon Variant Analyzer Software |
Fichou et al., Br J Haematol, 2014 (29) | RHD and RHCE; 34 additional blood groups | 4 | Commercially-available quality control DNA samples with known genotypes for 6 blood group systems | NA | Ion semiconductor sequencing | Mean read length 132 bp | Targeted: PCR amplification of exons, flanking introns and untranslated regions; specific targeted amplification of exons 1–2 of RHD and RHCE | Torrent Suite Software v4.0.2 and Variant Caller v3.4 |
Giollo et al., PLoS One, 2015 (30) | RHD described in detail, presumably RHCE included as well; 29 additional blood groups | 69 | Personal Genome Project data | NA | NA | NA | WGS | BOOGIE software developed for blood typing, infers the closest haplotype |
Lane et al., Transfusion, 2016 (31) | RHD and RHCE; 34 additional blood groups | 1 | MedSeq project | European | Sequencing by synthesis | 100 bp, paired-end | WGS | Burrows-Wheeler Aligner, Genomic Analysis Tool Kit |
Baronas et al., J Blood Disord Transfus, 2016 (32) | RHD | 37 | MedSeq project | European descent | Sequencing by synthesis | 100 bp, paired-end | WGS | Read depth analysis to determine RHD zygosity |
Möller et al., Blood Adv, 2016 (21) | RHD and RHCE; 41 additional blood group genes | 2,504 | 1000 Genomes Project | 1000 Genomes Project, data represents 26 populations worldwide and divided into 5 superpopulations | NA | NA | Low-coverage WGS and deep ES; trios available for phasing | Custom analytic pipeline and public database: Erythrogene |
Schoeman et al., Transfusion, 2017 (33) | RHD and RHCE; 27 additional blood groups and 2 transcription factors | 28 | Donor samples extensively phenotyped and genotyped | Selected with diverse genomic changes | Sequencing by synthesis | 150 bp, paired-end | ES | CLC Genomics Workbench software. Copy number analysis for RH C/c alleles and RH hybrids |
Dezan et al., Blood Cells Mol Dis, 2017 (34) | RHD and RHCE | 35 | Peripheral blood from SCD patients with unexplained Rh antibodies | SCD patients | Ion semiconductor sequencing | 188 bp mean read length for RHD; 196 bp mean read length for RHCE | Targeted | Custom workflow, Ion Reporter Software version 5.0 |
El Wafi et al., Vox Sang, 2017 (27) | RHD | 9 | Samples serologically negative for RhD, positive for at least 1 RHD exon | NA | Sequencing by synthesis | No specified | Targeted: PCR-amplification of RHD exons, part of the promoter and deep intronic sequences | Unspecified pipeline and ALAMUT software for prediction of splicing |
Chou et al., Blood Adv, 2017 (35) | RHD and RHCE | 54 | Alloimmunized and non-alloimmunized patients with sickle cell anemia enrolled in SWiTCH study | Sickle cell anemia patients | Sequencing by synthesis | NA | ES | Alignment with Burrows-Wheeler, AtlasSNP, Cassandra software |
Schoeman et al., Transfusion, 2018 (36) | RHD and RHCE; 27 additional blood groups and 2 transcription factors | 29 | Unresolved samples with complex serologic typing | Selected due to serologic typing complexity | Sequencing by synthesis | 150 bp, paired-end | ES | CLC Genomics Workbench software and copy number analysis. SIFT and PolyPhen-2 to predict effect of novel variants |
Lane et al., Lancet Haematol, 2018 (19) | RHD and RHCE; 11 additional blood group systems and 22 human platelet antigens | 310 | MedSeq study and INTERVAL trial | MedSeq: European (n=90); African (m=13), Asian (n=4), and Hispanic (n=4). INTERVAL study: 220 genomes of European ancestry | Sequencing by synthesis | 100 bp paired-end reads | WGS | Creation of automated antigen-typing algorithm: BloodTyper. Initial workflow with Genome Analysis Tool Kit. RHD zygosity and RHCE*C prediction through copy number analysis |
Tounsi et al., Blood Adv, 2019 (24) | RHD; in addition to RHAG in 2 samples | 69 | Donor blood, selected for diversity of serologic Rh phenotypes | Diverse serologic Rh phenotypes | Ion semiconductor sequencing | NA | Targeted (long range PCR) | Includes introns, the objective was to set full haplotypes |
Wheeler et al., Genet Med, 2019 (37) | RHD and RHCE | 2,854 | WHO reference DNA, Asian and Native American blood donors, African American samples from the Jackson Heart Study | 4 WHO references, 1,135 Asian and Native American blood donors, 1,715 African American samples from the Jackson Heart Study | Sequencing by synthesis | 100 bp paired-end reads for WHO and Asian/Native American blood donor samples; 150 bp paired-end for Jackson Heart Study | Targeted and WGS (Jackson Heart Study) | Custom analytic method to estimate paralog-specific copy number. GATK haplotype caller and haplotypephased; functional annotation through SeattleSeq Annotation |
Schoeman et al., Transfusion, 2019 (38) | RHD and RHCE; 35 additional blood groups and 2 transcription factors | 72 | Data generated by the Telethon Kids Institute | Western Desert Indigenous Australians | NA | NA | ES | Read depth/copy number variant analysis for RHCE*C and RHD zygosity. Impact of missense variants determined by meta-SNP |
Dinardo et al., Transfusion, 2019 (39) | RHD and RHCE | 54 | SCD patients with history of unexplained Rh-antibodies | Patients with SCD | Ion semiconductor sequencing | NA | Targeted: PCR amplification of exons, flanking introns | Custom workflow, Ion Reporter Software version 5.0 |
Lane et al., Transfusion, 2019 (40) | RHD and RHCE; 34 additional blood groups | 75 | MilSeq Project: Enabling Personalized Medicine through ES in the US Air Force | 12% Hispanic, 65.3% European, 6.6% African, 2.6% Asian, 2.6% European/Hispanic, 1.3% others | Sequencing by synthesis | NA, short-read | ES | Optimization of BloodTyper software for ES |
Halls et al., Vox Sang, 2020 (41) | RHD and RHCE | 22 | Archived DNA, samples with diverse genotypes | Samples selected to represent diverse RH alleles | NA | NA, short-read | WGS | Optimization of BloodTyper algorithm for complex and uncommon RH alleles through three strategies: read depth, split reads, and paired reads |
Chang et al., Blood Adv, 2020 (42) | RHD and RHCE | 881 | Peripheral blood from patients with SCD enrolled in Sickle Cell Clinical Research and Intervention Program | Patients with SCD | NA | Short, paired-end reads | WGS | RHtyper: cloud-based algorithm to predict RHD and RHCE alleles from WGS data. Considers sequence consistency, phase, coverage profiling and a circular binary segmentation algorithm |
Stef et al., Transfusion, 2020 (43) | RHD and RHCE | 278 | Samples with diverse genotypes | Samples with diverse genotypes | Sequencing by synthesis | 300 bp limit | Targeted: non-specific RH-consensus primers | Custom developed data analysis software |
Roulis et al., Transfusion, 2020 (44) | RHD and RHCE; 62 additional genes | 34 | RBC reference samples, including 23 with a range of well-characterized RH haplotypes and structural variants | NA | Sequencing by synthesis | Short read | Targeted: probe-hybridization capture | In-house command line software: Aztryx. CNV performed manually |
Tammi et al., Blood Adv, 2020 (45) | RHD | 35 | Serologically D-negative samples from the Finnish national prenatal screening program that were not resolved by SSP-PCR | Finnish prenatal patients | Ion semiconductor sequencing | NA | Targeted: long-range PCR | CLC Genomics Workbench |
Jadhao et al., EBioMedicine, 2022 (46) | RHD and RHCE; 35 additional blood group systems | 402 | Complex serology cases from Australian Red Cross LifeBlood, MedSeq project, and Indigenous Australian study participants | 58 complex serology cases from Australian Red Cross LifeBlood, 100 samples from MedSeq project, and 244 from Indigenous Australian participants | NA, short-read | NA | WGS, ES and targeted | RBCeq: bioinformatics algorithm to report clinically significant, rare and novel variants with potential significance. Web server with detailed reports and visualization. Includes CNV for Rh prediction |
Zhang et al., Am J Hum Genet, 2022 (26) | RHD and RHCE | 11 | Peripheral blood, 2 White individuals and 9 Black individuals with SCD | 2 White individuals and 9 Black individuals with SCD selected for RH allele diversity | Zero-mode waveguide long-read | Long read sequencing, average consensus read of 2.1–2.9 kb | Targeted | PAClindrome, customized pipeline with de-novo assembly |
Jadhao et al., Blood Adv, 2022 (47) | RHD and RHCE; 35 additional blood groups and 2 transcription factors | 2,796 | Healthy older Australians from the Medical Genome Reference Bank | Australians | NA | No specified | WGS | RBCeq: bioinformatics algorithm to report clinically significant, rare and novel variants with potential significance. Web server with detailed reports and visualization. Includes CNV for Rh prediction |
Jadhao et al., Transfusion, 2022 (48) | RHD and RHCE; 35 additional blood groups and 2 transcription factors | 189 | Peripheral blood from Tiwi Islander Indigenous Australians | Tiwi Islander Indigenous Australians | NA | No specified | WGS | RBCeq: bioinformatics algorithm to report clinically significant, rare and novel variants with potential significance. Web server with detailed reports and visualization. Includes CNV for Rh prediction |
Steiert et al., NAR Genomics and Bioinformatics, 2022 (49) | 35 blood group genes and 2 transcription factors | 16 | 7 Genome-in-a-Bottle reference samples, 8 research cohort from ITM and 1 from DZHK, Germany | Trio Ashkenazim Jewish, trio Han Chinese, 1 European ancestry, 9 unspecified | Zero-mode waveguide long-read | 5.9–6.8 kb | Target enrichment | Several tools for aligning and QC, DeepVariant and WhatsHap for phasing and variant calling, in-house software DeepBlood for blood group calling |
Tounsi et al., Clin Chem, 2022 (50) | RHD | 13 | 1 donor, 12 prenatal Serologically D-negative samples | Finnish prenatal patients | Nanopore embedded flow cells | reads length mode 10,450 bp | Long-range PCR and 1D native barcoding gDNA protocol | Custom workflow with several tools, IGV for visualization and CLC main workbench |
NGS, next-generation sequencing; NA, not available or not specified; PCR, polymerase chain reaction; WGS, whole-genome sequencing; ES, exome sequencing; SCD, sickle cell disease; WHO, World Health Organization; SNP, single-nucleotide polymorphism; RBC, red blood cell; CNV, copy number variant; SSP, single specific primer; ITM, Institute of Transfusion Medicine; DZHK, German Center for Cardiovascular Research; QC, quality control; gDNA, genomic DNA; IGV, integrative genomics viewer.
Copy number determination
One advantage of NGS technology over Sanger sequencing is its robust quantitative nature. The analysis of NGS outputs will not only provide a list of identified genomic variants, but also a copy number estimate for a given sequenced region. This facilitates the detection of duplications or deleted segments. For example, RHD weak D type 150 (RHD*01W.150) carries a duplication of exon 3, which is missed by array-based genotyping platforms but can be detected through NGS copy number analysis (43). In addition, the relatively short reads of some NGS chemistries may preclude unequivocal alignment of a sequenced fragment to the correct RH paralog gene. Examples include RHD exon 8, which is identical to RHCE exon 8 in the reference genome, and the RHCE allele that encodes for the C antigen, where the RHCE exon 2 sequence is identical to the reference version of its RHD counterpart. Figure 1 illustrates an example with the RHCE*CeRN allele (also known as RHCE*02.10.01), a clinically-relevant variant reported in 1% of individuals of African descent that results in partial C expression (23,51). The 2020 American Society of Hematology guidelines for transfusion support of sickle cell disease (SCD) patients recommend C− red cells for patients carrying an uncompensated RHCE*CeRN allele (52); accurate identification of this RHCE variant is therefore important to determine the optimal transfusion strategy for this patient group. Analytic approaches that employ copy number analysis to overcome the mis-alignment challenge and facilitate the detection of genomic rearrangement events in the RH locus are discussed below.
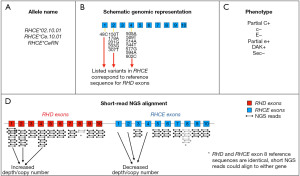
Haplotype determination
The wide variation of the RH locus raises another important consideration: many RH alleles are defined by multiple variants, which must all be present in the same haplotype to meet the genomic and phenotypic classification. The process of assigning genomic variants to specific haplotypes is commonly known as ‘phasing’. If hemizygosity of the RHD gene can be established, then phasing of all identified variants can be assumed with confidence in the final genotype and phenotype prediction. Tounsi et al. employed this approach to establish full RHD reference haplotypes with 69 hemizygous samples (24). However, when compound heterozygosity is a possibility, proper haplotype assignment may be critical to resolve the ambiguity and predict an accurate phenotype. Figure 2 illustrates an example of ambiguous genotyping for the Rh blood group. Short-read NGS technologies have limited phasing capabilities determined by the specific read length and frequency of overlapping heterozygosity. Longer read NGS platforms may overcome this specific limitation, improve mapping accuracy of sequenced fragments, and may permit de-novo assembly of the entire RH genomic locus (26,53).
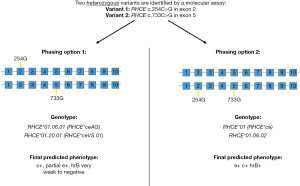
NGS chemistries and library preparation
Table 2 lists the type of NGS chemistry employed in peer-reviewed publications that include Rh blood group prediction. From a total of 28 studies, 11 (39.3%) employed sequencing-by-synthesis short-read NGS platforms, 5 (17.9%) employed ion semiconductor sequencing technology, 1 study used pyrosequencing, 2 employed zero-mode waveguide long-read chemistry and 1 employed nanopore embedded flow cell with long reads. The remaining studies do not specify the exact sequencing platform, but data suggests that all used short sequencing reads.
In terms of library preparation, whole-genome sequencing (WGS) is the most common approach to date (11 studies or 39.3%), followed by exome sequencing (ES) in 6 publications (21.4%) (Table 2). Some published studies employed more than one library preparation approach. Two custom-targeted NGS methods are currently represented in the Rh blood typing literature: polymerase chain reaction (PCR)-amplification (9 studies, 32.1%), and hybridization capture (3 publications). The advantages and limitations of the three main approaches (WGS, ES, and custom-target) have been discussed in depth elsewhere (8). The custom-target modality, designed to enrich for the specific genomic regions considered relevant for blood typing, reduces the possibility of secondary findings, an important ethical consideration discussed below.
One of the most promising NGS technologies is long read sequencing. Two main platforms are currently available in the market: zero-mode waveguide long read technology (Pacific Biosciences, Menlo Park, CA, USA) and nanopore embedded flow cells for ultra-long read sequencing (Oxford Nanopore Technologies, Oxford, UK) (53). The main contribution of these technologies would be the resolution of variant phasing, hybrid genes and other large structural variants, commonly found in the RHD/RHCE (26,49,50). Single long reads may cover long regions containing the variants, indels and break points that define the true allelic configuration of the haplotypes.
Sample or data sources
A unique feature of NGS data is that it can be stored long-term, to be re-analyzed and re-interpreted in the future when new genotype-phenotype knowledge becomes available. For example, if a new blood group gene were to be discovered, the laboratory could retrieve stored genomic sequences (provided they include the new locus of interest) and interpret the additional phenotype accordingly. With the rapidly growing number of RH alleles in electronic databases, it is conceivable that periodic re-interpretation of data incorporating all the recent genomic information will be a useful and cost-efficient practice. Indeed, transfusion medicine has been proposed as a universal application of clinical genomics (8), since any existing clinical sequencing dataset could potentially be re-analyzed for a precise and comprehensive blood type interpretation. Researchers have exploited the availability of worldwide sequencing cohorts to study blood typing applications, with 12 (42.9%) studies listed in Table 2 employing existing WGS or ES datasets as a sample source. Some of these are limited to analytic phenotype predictions, however, when a participant sample is not available for conventional serology or other forms of molecular blood antigen testing.
From the remaining studies, 18 (64.3%) used peripheral blood as a DNA sample source; six of them focused on blood donor samples and two on prenatal blood samples (45,50). Three studies utilized commercially available reference DNA samples to validate the specific NGS blood typing approach (29,37,49). Alternative tissue sources of DNA have not been cited for NGS blood group prediction in the literature, but they are feasible in the absence of transplantation, mosaicism, or genomic instability (such as in dysplasia or neoplasia). Interestingly, there is a scarcity of peer-reviewed literature on the use of RNA NGS for blood typing (54), although complementary DNA (cDNA) cloning and Sanger sequencing have been historically applied for resolution of hybrid events and phasing of multiple variants in RH transcripts (55).
Ethnoracial diversity
Transfusion literature has extensively documented that frequencies of blood group polymorphisms often correlate with an individual’s ethnoracial background or geographical descent (12,15,23). The Rh blood group is no exception, with variants described worldwide, including a large number of hybrid rearrangements and D− alleles described in individuals of African descent (13). Table 2 lists the ethnoracial composition, when available, for the blood samples or sequencing data analyzed in each study. From a grand total of 10,907 sequencing samples combined, four studies focused only on European samples (total n=86). Nine studies reported mixed race/ethnicity as detailed in Table 2 (total n=6,266). Four studies focused exclusively on samples from patients with a diagnosis of SCD (34,35,39,42), and an additional manuscript included nine samples from Black individuals with SCD (total n=998) (26). Four studies included samples from Indigenous peoples. One manuscript focused exclusively on Western Indigenous Australians (38) and a second manuscript on Tiwi Islander Indigenous Australians (48). Wheeler et al. analyzed 1,135 blood donor samples with Asian and Native American ancestry (37), and Jadhao et al. included 244 samples from Indigenous Australian participants (46). In five manuscripts, the selection of samples was performed to enrich for known genetic/serologic diversity or complexity (27,33,36,41,43).
Data analysis
The nucleic acid sequencing and data acquisition steps for blood typing purposes do not differ significantly from other clinical applications. Interpretation of the resulting data into a blood phenotype, however, requires transfusion medicine expertise and a tailored analytic workflow. Data analysis and storage, including the required information technology infrastructure, bioinformatics expertise, and software with associated source code, have been cited as the bottleneck for clinical genomics applications (5). Many studies listed in Table 2 describe the use of copy number analysis to resolve C antigen prediction and RH hybrid alleles. Nine custom analytic software for Rh blood typing are described currently in the literature. Giollo et al. created BOOGIE, a Boolean-based software that can infer haplotypes and is reported to have 100% accuracy for Rh+ (n=57) and 66% (n=12) for Rh− with full genome data from the Personal Genome Project (30). The Boolean phenotype interpretation rules of this software were based on the now retired BGMUT database (56). This was followed by the Erythrogene workflow, which details the analysis of the 1000 Genomes Project dataset for the prediction of known and novel alleles in 36 blood groups, resulting in the public Erythrogene database (21). Lane et al. developed the BloodTyper software and validated it with WGS data, with a subsequent release optimized for blood group predictions in ES (19,31,40). A third release of this algorithm was optimized specifically for complex RH haplotypes, employing read depth, split reads, and paired read analysis from WGS to achieve increased accuracy (32,41).
Two cloud-based analytic tools for Rh blood group prediction are described in the literature and publicly available. Chang et al. (42) released RHtyper for determination of RHD and RHCE haplotypes from WGS data, and Jadhao et al. (46) developed RBCeq to predict novel and known variants in 36 blood groups. The latter was also employed for blood group secondary analysis of existing WGS datasets from Indigenous Tiwi Islander and healthy older Australian cohorts, revealing novel RH variants in both instances (47,48). Other unique, quantitative analysis algorithms specific for the RH locus were described by Wheeler et al. (37) and Stef et al. (43), the former validated with WGS and targeted NGS data, and the latter applied to amplicons generated with non-specific RH consensus primers. Roulis et al. describe a command-line software, Aztryx, to interpret a probe-capture targeted dataset for 64 red cell, platelet, and neutrophil antigen genes (44).
The algorithms described above have been validated with short-read sequencing datasets. Three peer-reviewed study employing long-read NGS technology for the RH locus are currently available in the literature. Zhang et al. describe the PAClindrome analysis pipeline to derive consensus sequences of 2.1–2.9 kb average length, allowing for physical phasing of contiguous fragments and for de-novo assembly of the RH locus in four participants of African descent (26).
Comparison with other blood typing methods
Regardless of the advantages in cost and throughput, equivalent or superior accuracy compared to standard blood typing methods is an indispensable requirement for broad adoption of new molecular antigen typing technologies. Publications available range from bioinformatic predictions only, to broad validations involving serologic phenotyping, targeted SNV-based typing arrays, Sanger sequencing of genomic DNA or of cloned plasmids, quantitative multiplex PCR of short fluorescent fragments, allele-specific PCR, PCR-based hybrid box zygosity assays, and long-range PCR. The type and number of orthogonal validations varies by study, depending on the availability of samples, comparison data, scope, and goals of the project. Comparisons with molecular data in earlier publications fall in the 90–95% accuracy range (28,30), but recent optimized analytic methods cite accuracies >99.5% (19,37,40,41). A common reported finding in multiple studies is that NGS identifies additional variants that were not detected by SNV-based typing arrays (21,26,33,34,36,38,41,42,45,47,48), therefore providing more accuracy or detecting novel variants in those instances. Chang et al. describe two cases of RHD hemizygosity that were missed by the PCR-based zygosity test, as well as a variant that was missed by Sanger sequencing but detected by NGS, presumably due to variations that interfered with Sanger sequencing primer binding (42). Dezan et al. reported that in 42 patients with abnormal Rh antibodies, 62% were determined to be incorrectly classified by serological methods as auto/allospecific after reviewing NGS data (34). They also describe 9 cases that had been incorrectly classified as partial D and that were transitioned to a D+ transfusion strategy after NGS results became available, leading to 10% lower usage of D− units in the inventory (34). Zhang et al. describe that long-read NGS had higher detection of microconversions and more accurate determination of breakpoints, and it resolved RHD-RHCE haplotype linkage in two cases that had been inferred incorrectly by known frequencies (26). Steiert et al. better elucidated the true allelic configuration for a heterozygous RHCE alleles, corrected a structural variation caused by misalignments of short reads and detected an insertion that could not be detected in the short-read dataset (49).
Clinical scenarios with dual genomic populations
As discussed previously, NGS outputs are quantitative and permit copy number determinations. Therefore, physiologic or pathologic states that result in dual genomic populations affecting blood group loci could be discerned by NGS blood typing methods. For example, myeloid dysplastic/neoplastic states have been associated with clonal progenitor loss of heterozygosity in chromosome 1p and a resulting acquired genomic and phenotypic mosaicism for the Rh blood group (57-59). These instances may be overlooked by current automated blood typing platforms, but NGS methods possess the sensitivity to detect any cases with unexpected allele fractions. Schoeman et al. reported a minor genomic population encoding for D, C, and e antigens in a sample demonstrating mixed-field reactions with anti-D, anti-C, and anti-e (36). Another scenario involving dual populations is the hematopoietic progenitor cell post-transplantation engraftment period, but no studies have explored the utility of RH NGS blood typing in this setting or its correlation with the circulating red blood cell phenotype.
Non-invasive prenatal testing (NIPT) also requires sensitive detection of a minor genomic population, in this case fragmented cell-free fetal DNA. In a growing number of countries, NIPT has been adopted to screen non-immunized pregnant patients for targeted RhIg prophylaxis (60-64). The use of NGS for blood group NIPT has been discussed in the literature and demonstrated with Rh and other blood group and platelet antigens (65-68). Although these publications describe a small number of samples, they show the feasibility of applying targeted amplicon-based NGS technology to the field. Issues that a NGS approach can resolve include the identification of RHD-positive, antigen negative alleles (69) and presence of fetal DNA (70). Still, more work is required to determine the interpretation criteria of multiple factors, such as cut-off ratios of fetal DNA presence, correct interpretation of copy numbers, and fetal allele calling, among others (71).
Social, ethical, and legal considerations for NGS in blood typing
NGS has the potential to advance transfusion medicine and help provide individualized care for patients (72). Blood operators pursuing NGS for red cell antigen typing must consider the social, cultural, ethical, and legal implications of this technology. NGS should be used in a way that allows everyone, regardless of their socio-economic status, ethnicity, or gender identity to benefit from it (73). Beyond advancing scientific knowledge, NGS testing should also consider the value to those who are being tested.
Donor perspectives on genomic red cell antigen testing have been recently studied (74-76). In a recent study, genotyping results were provided with accompanying educational material, recommendations for healthcare provider follow-up, and access to individual counselling (74). This comprehensive reporting format seems valuable; however, there is a lack of participant reported outcomes and long-term follow-up data. Today, red cell antigen genotyping is routinely performed to support transfusion of patients. Focus group-based study on perceptions of red cell antigen genotyping found that participants felt the testing was appropriate in special circumstances where it can directly impact transfusion practice (75). However, the use of donor samples to test for additional markers relating to iron metabolism and bowel cancer invoked concerns of long-term health-related impact, personal health data privacy and security, and health insurance implications. Healthcare practitioners asked about extensive donor genotyping also expressed concerns regarding data security and privacy (76). These studies highlighted the need for tailored informed consent when novel and more extensive genetic testing is implemented.
For NGS analysis of the Rh system, information regarding ethnicity, race or geographic origin may be limited (Table 2). However, whether this information has value to the people being studied must be established with stakeholders, ensuring the benefits are meaningful and accessible to the individuals being tested. Historically, scientific inquiry has disproportionately exposed individuals from specific racial groups and disadvantaged socio-economic status, by enrolling vulnerable individuals into studies that have potential for greater harm and excluding the same populations from accessing the data and preventing them to benefit from their participation (77,78). Stakeholder input should be sought during planning and implementation of NGS to ensure inclusion of all and clarify the best approach for obtaining informed consent from donors and patients. Seeking permission from Indigenous councils is a legal requirement in some jurisdictions when testing individuals from Indigenous populations (79).
When embarking on NGS testing, a “living library” of data would be generated. This data may include actionable secondary findings of importance to the individual (80-82). There is ambivalence in the literature regarding which findings may be considered actionable. Hence, for NGS of red cell antigens, blood operators may need input from the populations they serve and consult guidelines regarding the secondary findings that must be disclosed. Moreover, the testing authority may need to provide guidance regarding the health, employment and/or insurance related implications of the secondary information that is conveyed (83). Secondary findings can be partly reduced by limiting the genes interrogated by NGS.
The vast amount of biological data generated with NGS is considered personal health-related information. Factors that influence which legislation would be applied to the NGS data depends on who collects, stores, and analyzes the information and its intended use. There exists jurisdictional variation in how this information is protected to ensure individual’s privacy (84-86). The privacy law governing NGS data may also change over time as the context in which testing is conducted or used changes. Hence, NGS implementation by blood operators requires consultation with legal counsel, privacy experts and bioethicists to ensure appropriate measures are in place to protect the information.
Future outlook
The growth of blood typing genomics programs will likely continue to unravel the full diversity of the RH genomic locus worldwide. Alloimmunization in patients with hemoglobinopathy has been documented in spite of conventional Rh phenotype blood matching, and RH genotype-matching for transfusions has been proposed as a strategy to better prevent alloimmunization in this frequently-transfused group (87-92). NGS technologies continue to evolve rapidly, with superior and/or more affordable chemistries and platforms periodically entering the market, and more likely in the horizon. As genomics becomes progressively more attainable and affordable for blood operators, the possibility of precise molecular Rh blood group characterization for an entire donor base, enabling new high-resolution blood allocation algorithms, may come closer to fruition.
Conclusions
The application of NGS technology in immunohematology provides the high genetic resolution and throughput required for optimal RH genotyping, patient-donor matching, and transfusion care. This technical revolution must be paralleled by legal and ethical safeguards for data use. Discussions regarding patient consent, secondary findings, health equity, and increased/future data analysis should occur simultaneously with the development of laboratory methods to guarantee the success of genomics programs. RH genotyping through NGS has the potential to significantly advance the transfusion medicine field.
Acknowledgments
Funding: None.
Footnote
Provenance and Peer Review: This article was commissioned by the Guest Editor (Lilian Castilho) for the series “Serology and Molecular Biology of the Rh System” published in Annals of Blood. The article has undergone external peer review.
Peer Review File: Available at https://aob.amegroups.com/article/view/10.21037/aob-23-10/prf
Conflicts of Interest: All authors have completed the ICMJE uniform disclosure form (available at https://aob.amegroups.com/article/view/10.21037/aob-23-10/coif). The series “Serology and Molecular Biology of the Rh System” was commissioned by the editorial office without any funding or sponsorship. CM reports one-time paid consultancy in October-November 2021 from ISBT RCIBGT Working Party consultant, and honoraria for Grand Rounds Lecture from Tufts Medical Center. CM also reports Fiduciary duty as member of the Board of Directors of the AABB. The authors have no other conflicts of interest to declare.
Ethical Statement: The authors are accountable for all aspects of the work in ensuring that questions related to the accuracy or integrity of any part of the work are appropriately investigated and resolved.
Open Access Statement: This is an Open Access article distributed in accordance with the Creative Commons Attribution-NonCommercial-NoDerivs 4.0 International License (CC BY-NC-ND 4.0), which permits the non-commercial replication and distribution of the article with the strict proviso that no changes or edits are made and the original work is properly cited (including links to both the formal publication through the relevant DOI and the license). See: https://creativecommons.org/licenses/by-nc-nd/4.0/.
References
- Slatko BE, Gardner AF, Ausubel FM. Overview of Next-Generation Sequencing Technologies. Curr Protoc Mol Biol 2018;122:e59. [Crossref] [PubMed]
- 1000 Genomes Project Consortium; Auton A, Brooks LD, et al. A global reference for human genetic variation. Nature 2015;526:68-74.
- NHGRI. The Cost of Sequencing a Human Genome. Available online: https://www.genome.gov/about-genomics/fact-sheets/Sequencing-Human-Genome-cost
- Collins FS, Varmus H. A new initiative on precision medicine. N Engl J Med 2015;372:793-5. [Crossref] [PubMed]
- Suwinski P, Ong C, Ling MHT, et al. Advancing Personalized Medicine Through the Application of Whole Exome Sequencing and Big Data Analytics. Front Genet 2019;10:49. [Crossref] [PubMed]
- Profaizer T, Lázár-Molnár E, Close DW, et al. HLA genotyping in the clinical laboratory: comparison of next-generation sequencing methods. HLA 2016;88:14-24. [Crossref] [PubMed]
- Lane WJ. Recent advances in blood group genotyping. Ann Blood 2021;6:31. [Crossref]
- Montemayor C, Brunker PAR, Keller MA. Banking with precision: transfusion medicine as a potential universal application in clinical genomics. Curr Opin Hematol 2019;26:480-7. [Crossref] [PubMed]
- Hyland CA, Roulis EV, Schoeman EM. Developments beyond blood group serology in the genomics era. Br J Haematol 2019;184:897-911. [Crossref] [PubMed]
- Wheeler MM, Johnsen JM. The role of genomics in transfusion medicine. Curr Opin Hematol 2018;25:509-15. [Crossref] [PubMed]
- Cone Sullivan JK, Gleadall N, Lane WJ. Blood Group Genotyping. Clin Lab Med 2022;42:645-68. [Crossref] [PubMed]
- Cohn CS, Delaney M, Johnson ST, et al. editors. Technical Manual. 20th ed. Bethesda: AABB; 2020.
- Fichou Y. Hybrids and microconversions in RH genes: investigation and implication in transfusion therapy. Ann Blood 2023;8:7. [Crossref]
- Floch A. Molecular genetics of the Rh blood group system: alleles and antibodies—a narrative review. Ann Blood 2021;6:29. [Crossref]
- Daniels G. Human Blood Groups. 3rd ed. West Sussex: Wiley-Blackwell; 2013.
- ISBT. Red Cell Immunogenetics and Blood Group Terminology. Available online: https://www.isbtweb.org/working-parties/red-cell-immunogenetics-and-blood-group-terminology/
- Alattar AG, Storry JR, Olsson ML. Challenging the definition of a blood group: Proposal to make CD36 a novel blood group system based on a case report and pre-existing genetic, proteomic and clinical data. In: 33rd Regional ISBT Congress. Gothenburg: VoxSanguinis; 2023:81-2.
- Wagner FF, Flegel WA. The Rhesus Site. Transfus Med Hemother 2014;41:357-63. [Crossref] [PubMed]
- Lane WJ, Westhoff CM, Gleadall NS, et al. Automated typing of red blood cell and platelet antigens: a whole-genome sequencing study. Lancet Haematol 2018;5:e241-51. [Crossref] [PubMed]
- Rophina M, Pandhare K, Jadhao S, et al. BGvar: A comprehensive resource for blood group immunogenetics. Transfus Med 2022;32:229-36. [Crossref] [PubMed]
- Möller M, Jöud M, Storry JR, et al. Erythrogene: a database for in-depth analysis of the extensive variation in 36 blood group systems in the 1000 Genomes Project. Blood Adv 2016;1:240-9. [Crossref] [PubMed]
- Floch A, Téletchéa S, Tournamille C, et al. A Review of the Literature Organized Into a New Database: RHeference. Transfus Med Rev 2021;35:70-7. [Crossref] [PubMed]
- Reid ME, Lomas-Francis C, Olsson ML. The blood group antigen factsbook. 3rd ed. London: Elsevier; 2012.
- Tounsi WA, Madgett TE, Avent ND. Complete RHD next-generation sequencing: establishment of reference RHD alleles. Blood Adv 2018;2:2713-23. Erratum in: Blood Adv 2019;3:120. [Crossref] [PubMed]
- Wagner FF, Frohmajer A, Flegel WA. RHD positive haplotypes in D negative Europeans. BMC Genet 2001;2:10. [Crossref] [PubMed]
- Zhang Z, An HH, Vege S, et al. Accurate long-read sequencing allows assembly of the duplicated RHD and RHCE genes harboring variants relevant to blood transfusion. Am J Hum Genet 2022;109:180-91. [Crossref] [PubMed]
- El Wafi M, El Housse H, Zaid N, et al. Novel intronic RHD variants identified in serologically D-negative blood donors. Vox Sang 2017;112:796-802. [Crossref] [PubMed]
- Stabentheiner S, Danzer M, Niklas N, et al. Overcoming methodical limits of standard RHD genotyping by next-generation sequencing. Vox Sang 2011;100:381-8. [Crossref] [PubMed]
- Fichou Y, Audrézet MP, Guéguen P, et al. Next-generation sequencing is a credible strategy for blood group genotyping. Br J Haematol 2014;167:554-62. [Crossref] [PubMed]
- Giollo M, Minervini G, Scalzotto M, et al. BOOGIE: Predicting Blood Groups from High Throughput Sequencing Data. PLoS One 2015;10:e0124579. [Crossref] [PubMed]
- Lane WJ, Westhoff CM, Uy JM, et al. Comprehensive red blood cell and platelet antigen prediction from whole genome sequencing: proof of principle. Transfusion 2016;56:743-54. [Crossref] [PubMed]
- Baronas J, Westhoff CM, Vege S, et al. RHD zygosity determination from whole genome sequencing data. J Blood Disord Transfus 2016;7:5. [Crossref]
- Schoeman EM, Lopez GH, McGowan EC, et al. Evaluation of targeted exome sequencing for 28 protein-based blood group systems, including the homologous gene systems, for blood group genotyping. Transfusion 2017;57:1078-88. [Crossref] [PubMed]
- Dezan MR, Ribeiro IH, Oliveira VB, et al. RHD and RHCE genotyping by next-generation sequencing is an effective strategy to identify molecular variants within sickle cell disease patients. Blood Cells Mol Dis 2017;65:8-15. [Crossref] [PubMed]
- Chou ST, Flanagan JM, Vege S, et al. Whole-exome sequencing for RH genotyping and alloimmunization risk in children with sickle cell anemia. Blood Adv 2017;1:1414-22. [Crossref] [PubMed]
- Schoeman EM, Roulis EV, Liew YW, et al. Targeted exome sequencing defines novel and rare variants in complex blood group serology cases for a red blood cell reference laboratory setting. Transfusion 2018;58:284-93. [Crossref] [PubMed]
- Wheeler MM, Lannert KW, Huston H, et al. Genomic characterization of the RH locus detects complex and novel structural variation in multi-ethnic cohorts. Genet Med 2019;21:477-86. [Crossref] [PubMed]
- Schoeman EM, Roulis EV, Perry MA, et al. Comprehensive blood group antigen profile predictions for Western Desert Indigenous Australians from whole exome sequence data. Transfusion 2019;59:768-78. [Crossref] [PubMed]
- Dinardo CL, Kelly S, Dezan MR, et al. Diversity of RH and transfusion support in Brazilian sickle cell disease patients with unexplained Rh antibodies. Transfusion 2019;59:3228-35. [Crossref] [PubMed]
- Lane WJ, Vege S, Mah HH, et al. Automated typing of red blood cell and platelet antigens from whole exome sequences. Transfusion 2019;59:3253-63. [Crossref] [PubMed]
- Halls JBL, Vege S, Simmons DP, et al. Overcoming the challenges of interpreting complex and uncommon RH alleles from whole genomes. Vox Sang 2020;115:790-801. [Crossref] [PubMed]
- Chang TC, Haupfear KM, Yu J, et al. A novel algorithm comprehensively characterizes human RH genes using whole-genome sequencing data. Blood Adv 2020;4:4347-57. [Crossref] [PubMed]
- Stef M, Fennell K, Apraiz I, et al. RH genotyping by nonspecific quantitative next-generation sequencing. Transfusion 2020;60:2691-701. [Crossref] [PubMed]
- Roulis E, Schoeman E, Hobbs M, et al. Targeted exome sequencing designed for blood group, platelet, and neutrophil antigen investigations: Proof-of-principle study for a customized single-test system. Transfusion 2020;60:2108-20. [Crossref] [PubMed]
- Tammi SM, Tounsi WA, Sainio S, et al. Next-generation sequencing of 35 RHD variants in 16 253 serologically D- pregnant women in the Finnish population. Blood Adv 2020;4:4994-5001. [Crossref] [PubMed]
- Jadhao S, Davison CL, Roulis EV, et al. RBCeq: A robust and scalable algorithm for accurate genetic blood typing. EBioMedicine 2022;76:103759. [Crossref] [PubMed]
- Jadhao S, Davison C, Roulis EV, et al. Using whole-genome sequencing to characterize clinically significant blood groups among healthy older Australians. Blood Adv 2022;6:4593-604. [Crossref] [PubMed]
- Jadhao S, Hoy W, Lee S, et al. The genomic landscape of blood groups in Indigenous Australians in remote communities. Transfusion 2022;62:1110-20. [Crossref] [PubMed]
- Steiert TA, Fuß J, Juzenas S, et al. High-throughput method for the hybridisation-based targeted enrichment of long genomic fragments for PacBio third-generation sequencing. NAR Genom Bioinform 2022;4:lqac051. [Crossref] [PubMed]
- Tounsi WA, Lenis VP, Tammi SM, et al. Rh Blood Group D Antigen Genotyping Using a Portable Nanopore-based Sequencing Device: Proof of Principle. Clin Chem 2022;68:1196-201. [Crossref] [PubMed]
- Rouillac C, Gane P, Cartron J, et al. Molecular basis of the altered antigenic expression of RhD in weak D(Du) and RhC/e in RN phenotypes. Blood 1996;87:4853-61. [Crossref] [PubMed]
- Chou ST, Alsawas M, Fasano RM, et al. American Society of Hematology 2020 guidelines for sickle cell disease: transfusion support. Blood Adv 2020;4:327-55. [Crossref] [PubMed]
- Thun GA, Gueuning M, Mattle-Greminger M. Long-read sequencing in blood group genetics. Transfusion Medicine and Hemotherapy 2023;50:184-97. [Crossref]
- Ji Y, Luo Y, Wen J, et al. Patients with Asian-type DEL can safely be transfused with RhD-positive blood. Blood 2023;141:2141-50. [Crossref] [PubMed]
- Rouillac C, Colin Y, Hughes-Jones NC, et al. Transcript analysis of D category phenotypes predicts hybrid Rh D-CE-D proteins associated with alteration of D epitopes. Blood 1995;85:2937-44. [Crossref] [PubMed]
- Patnaik SK, Helmberg W, Blumenfeld OO. BGMUT: NCBI dbRBC database of allelic variations of genes encoding antigens of blood group systems. Nucleic Acids Res 2012;40:D1023-9. [Crossref] [PubMed]
- Montemayor-Garcia C, Coward R, Albitar M, et al. Acquired RhD mosaicism identifies fibrotic transformation of thrombopoietin receptor-mutated essential thrombocythemia. Transfusion 2017;57:2136-9. [Crossref] [PubMed]
- Körmöczi GF, Dauber EM, Haas OA, et al. Mosaicism due to myeloid lineage restricted loss of heterozygosity as cause of spontaneous Rh phenotype splitting. Blood 2007;110:2148-57. [Crossref] [PubMed]
- Orlando N, Putzulu R, Nuzzolo ER, et al. Primary myelofibrosis: when the clone manifests with Rh phenotype splitting. Ann Hematol 2014;93:1077-8. [Crossref] [PubMed]
- Minon JM, Gerard C, Senterre JM, et al. Routine fetal RHD genotyping with maternal plasma: a four-year experience in Belgium. Transfusion 2008;48:373-81. [Crossref] [PubMed]
- Clausen FB, Steffensen R, Christiansen M, et al. Routine noninvasive prenatal screening for fetal RHD in plasma of RhD-negative pregnant women-2 years of screening experience from Denmark. Prenat Diagn 2014;34:1000-5. [Crossref] [PubMed]
- Scheffer PG, Ait Soussan A, Verhagen OJ, et al. Noninvasive fetal genotyping of human platelet antigen-1a. BJOG 2011;118:1392-5. [Crossref] [PubMed]
- de Haas M, Thurik FF, van der Ploeg CP, et al. Sensitivity of fetal RHD screening for safe guidance of targeted anti-D immunoglobulin prophylaxis: prospective cohort study of a nationwide programme in the Netherlands. BMJ 2016;355:i5789. [Crossref] [PubMed]
- Haimila K, Sulin K, Kuosmanen M, et al. Targeted antenatal anti-D prophylaxis program for RhD-negative pregnant women - outcome of the first two years of a national program in Finland. Acta Obstet Gynecol Scand 2017;96:1228-33. [Crossref] [PubMed]
- Rieneck K, Bak M, Jønson L, et al. Next-generation sequencing: proof of concept for antenatal prediction of the fetal Kell blood group phenotype from cell-free fetal DNA in maternal plasma. Transfusion 2013;53:2892-8. [Crossref] [PubMed]
- Orzińska A, Guz K, Mikula M, et al. Prediction of fetal blood group and platelet antigens from maternal plasma using next-generation sequencing. Transfusion 2019;59:1102-7. [Crossref] [PubMed]
- Orzińska A, Guz K, Uhrynowska M, et al. Noninvasive prenatal HPA-1 typing in HPA-1a negative pregnancies selected in the Polish PREVFNAIT screening program. Transfusion 2018;58:2705-11. [Crossref] [PubMed]
- Rieneck K, Clausen FB, Bergholt T, et al. Prenatal prediction of fetal Rh C, c and E status by amplification of maternal cfDNA and deep sequencing. Prenat Diagn 2021;41:1380-8. [Crossref] [PubMed]
- Takahashi K, Migita O, Sasaki A, et al. Amplicon Sequencing-Based Noninvasive Fetal Genotyping for RHD-Positive D Antigen-Negative Alleles. Clin Chem 2019;65:1307-16. [Crossref] [PubMed]
- Wienzek-Lischka S, Krautwurst A, Fröhner V, et al. Noninvasive fetal genotyping of human platelet antigen-1a using targeted massively parallel sequencing. Transfusion 2015;55:1538-44. [Crossref] [PubMed]
- Wienzek-Lischka S, Bachmann S, Froehner V, et al. Potential of Next-Generation Sequencing in Noninvasive Fetal Molecular Blood Group Genotyping. Transfus Med Hemother 2020;47:14-22. [Crossref] [PubMed]
- Fichou Y, Férec C. NGS and blood group systems: State of the art and perspectives. Transfus Clin Biol 2017;24:240-4. [Crossref] [PubMed]
- Wilhelm E, Ballalai I, Belanger ME, et al. Measuring the Burden of Infodemics: Summary of the Methods and Results of the Fifth WHO Infodemic Management Conference. JMIR Infodemiology 2023;3:e44207. [Crossref] [PubMed]
- Casalino S, Frangione E, Chung M, et al. Genome screening, reporting, and genetic counseling for healthy populations. Hum Genet 2023;142:181-92. [Crossref] [PubMed]
- Thorpe R, Jensen K, Masser B, et al. Donor and non-donor perspectives on receiving information from routine genomic testing of donor blood. Transfusion 2023;63:331-8. [Crossref] [PubMed]
- Luken JS, Ritsema SP, Van der Wal MM, et al. Mapping anticipated advantages and disadvantages of implementation of extensive donor genotyping: A focus group approach. Transfus Med 2022;32:366-74. [Crossref] [PubMed]
- Raven-Gregg T, Shepherd V. Exploring the inclusion of under-served groups in trials methodology research: an example from ethnic minority populations' views on deferred consent. Trials 2021;22:589. [Crossref] [PubMed]
- Hussain-Gambles M. Ethnic minority under-representation in clinical trials. Whose responsibility is it anyway? J Health Organ Manag 2003;17:138-43. [Crossref] [PubMed]
- FNIGC. The First Nations Principles of OCAP®. Available online: https://fnigc.ca/ocap-training/
- Chassagne A, Pélissier A, Houdayer F, et al. Exome sequencing in clinical settings: preferences and experiences of parents of children with rare diseases (SEQUAPRE study). Eur J Hum Genet 2019;27:701-10. [Crossref] [PubMed]
- Houdayer F, Putois O, Babonneau ML, et al. Secondary findings from next generation sequencing: Psychological and ethical issues. Family and patient perspectives. Eur J Med Genet 2019;62:103711. [Crossref] [PubMed]
- Biesecker BB, Klein W, Lewis KL, et al. How do research participants perceive "uncertainty" in genome sequencing? Genet Med 2014;16:977-80. [Crossref] [PubMed]
- The Panel on Research Ethics. TCPS 2 (2022)—Chapter 13: Human Genetic Research. Available online: https://ethics.gc.ca/eng/tcps2-eptc2_2022_chapter13-chapitre13.html
- ASPE. Health Insurance Portability and Accountability Act of 1996. (Updated Aug 20, 1996). Available online: https://aspe.hhs.gov/reports/health-insurance-portability-accountability-act-1996
- Office of the Privacy Commissioner of Canada. The Personal Information Protection and Electronic Documents Act (PIPEDA). (Updated 2021-12-08). Available online: https://www.priv.gc.ca/en/privacy-topics/privacy-laws-in-canada/the-personal-information-protection-and-electronic-documents-act-pipeda/
- e-Laws. Personal Health Information Protection Act, 2004, S.O. 2004, c. 3, Sched. A. Available online: https://www.ontario.ca/laws/statute/04p03
- Takasaki K, Friedman DF, Uter S, et al. Variant RHD alleles and Rh immunization in patients with sickle cell disease. Br J Haematol 2023;201:1220-8. [Crossref] [PubMed]
- Waldis SJ, Uter S, Kavitsky D, et al. Rh alloimmunization in chronically transfused patients with thalassemia receiving RhD, C, E, and K matched transfusions. Blood Adv 2021;5:737-44. [Crossref] [PubMed]
- Chou ST, Evans P, Vege S, et al. RH genotype matching for transfusion support in sickle cell disease. Blood 2018;132:1198-207. [Crossref] [PubMed]
- Chou ST, Jackson T, Vege S, et al. High prevalence of red blood cell alloimmunization in sickle cell disease despite transfusion from Rh-matched minority donors. Blood 2013;122:1062-71. [Crossref] [PubMed]
- da Costa DC, Pellegrino J Jr, Guelsin GA, et al. Molecular matching of red blood cells is superior to serological matching in sickle cell disease patients. Rev Bras Hematol Hemoter 2013;35:35-8. [Crossref] [PubMed]
- Castilho L, Dinardo CL. Optimized Antigen-Matched in Sickle Cell Disease Patients: Chances and Challenges in Molecular Times - the Brazilian Way. Transfus Med Hemother 2018;45:258-62. [Crossref] [PubMed]
Cite this article as: Khandelwal A, Zittermann S, Sierocinski T, Montemayor C. RH genotyping by next-generation sequencing. Ann Blood 2023;8:39.