Correlation analyses between age and indices in routine blood laboratory tests suggest potential aging biomarkers
Introduction
Population aging is a worldwide socioeconomic issue and has a great impact on the health care system. From a biological perspective, aging is a natural, multi-factorial phenomenon characterized by the accumulation of degradation processes, which in turn are supported by multiple changes and damages within molecular pathways (1). Such changes and damages will eventually impair the functions of cells and tissues. Therefore, aging is the most serious risk factor for almost all noncommunicable diseases, including cardiovascular diseases, tumors, diabetes and neurological diseases.
With the aggravation of population aging, there is a great interest in determining the predictors, that is, biomarkers of population aging. The study on biomarkers, which refers to the measurements of cellular, biochemical, or molecular changes, has shown significant value in health prediction in humans. Several aging clocks have been proposed that use various biomarkers to predict the actual physiological age of humans (2). Currently, the most accurate markers reported are methylated markers used in prediction models for muscle aging (3), while the transcription and metabolomics are not (4). Although many theories have been put forward to explain the phenomenon of aging, the lack of sensitive and specific biomarkers has been a great hurdle to predicting human aging (5). Recent attempts to use techniques such as principal component analysis to statistically link multiple biomarkers running in different physiological networks have led to a more comprehensive understanding of age-related health (6). The transition from a simple method based on one biomarker at a time to a systematic analysis method that integrates multiple biomarkers simultaneously provides an opportunity to identify the comprehensive biomarkers specific to aging (7). Therefore, discovering biomarkers that can predict disease occurrence remains an important goal.
The decline of the immune system, known as immune senescence, includes a series of changes in the innate and adaptive immune systems, and is accompanied with human aging. These result in increased susceptibility to infections, reduce the efficacy of vaccination, and increase the incidence of tumors, neurodegenerative diseases and metabolic imbalances in the elderly (8). In order to meet the health-related needs of the growing elderly population, the study on age-related immune aging needs to be developed rapidly. In addition, in order to develop appropriate preventive and therapeutic strategies, we must understand the potential biological mechanisms that lead to age-related pathogenesis (9). In this study, the differences of indices from routine blood laboratory tests, including Complete Blood Count, blood biochemistry panel were compared among different age groups. Furthermore, we analyzed the differences of immune cells and inflammatory cytokines, as well as the differences in gene expression levels among different age groups. Our results showed significant correlations between some indices with aging, and some might even be associated with genders and ethnics. Our work provides clues to look for potential biomarkers of aging in the future. We present the following article in accordance with the MDAR reporting checklist (available at https://aob.amegroups.com/article/view/10.21037/aob-20-79/rc).
Methods
Data sources
Data sources for this article are available at http://10kimmunomes.org/. Here, we proposed the 10,000 immune bodies project (10KIP), which was a framework to develop multiple human immune system references from the ImmPort, a publicly available source of immune system data. Although some measurement types were sparse in the existing immune port databases, the existing data allowed multiple robustness comparisons. The study was conducted in accordance with the Declaration of Helsinki (as revised in 2013).
Statistical analysis
Data management and statistical analysis were performed by using IBM SPSS version 25 (IBM, Armonk, NY, USA). Spearman rank correlation was used to calculate the correlation between biomarkers and age, sex and race. Nonparametric test was used to calculate the differences among different age groups. Graphic representation was performed by using GraphPad Prism 7.0.
Differentially expressed genes (DEGs) analysis
Statistical analyses were carried out by using R3.6.1 and Bioconductor (http://www.bioconductor.org/). Selection criteria were |log2FC|>0.5 and P<0.001.
Functional analysis of DEGs
Using DAVID (https:/david.ncifcrf.gov/) to analyze DEGs. The five most significant functions of DEG enrichment were selected for analysis.
Protein-protein interaction networks
In this study, the interaction network diagram of target genes coding proteins was drawn by STRING database in order to understand the mutual regulation relationship between DEGs.
Results
Correlations between aging and indices in cell population and blood tests
To identify potential biomarkers related to aging, the correlations between aging and different indices were analyzed, including 24 cell population indices from 562 persons, 78 cytokines from 952 persons, 19 Complete Blood Count indices from 1,155 persons, 7 blood lipids and 18 other indices in blood chemistry panel from 664 persons (Tables 1-5). Coefficients of Spearman Rank Correlation were calculated and the results were shown in Figure 1. Among cell populations, Naive CD8 T cells, Naive CD4 T cells, Naive B cells, B cells and Transitional B cells showed negative correlations to aging, while NK cells, Central Memory CD4 T cells, Central Memory CD8 T cells and Monocytes showed positive correlations to aging (Figure 1A). Meanwhile, cytokines CCL22 and IL21 showed negative correlations to aging, while CXCL8 and CXCL9 showed positive correlations to aging (Figure 1B). As seen in Figure 1C, mean corpuscular hemoglobin concentration (MCHC) (r=–0.044, P=0.138) and platelet count (r=–0.143, P<0.001) showed negative correlations to aging. In terms of blood chemistry panel, total cholesterol and non-high-density lipoprotein (HDL) cholesterol calculation showed negative correlations to aging (Figure 1D), while urea nitrogen and albumin showed positive correlations to aging (Figure 1E).
Table 1
Cell population | All (n=562) | Young (n=193) | Middle-aged (n=113) | Elder (n=256) | P value |
---|---|---|---|---|---|
Age, years | 55.81 (30.35, 77.25) | 26.5 (22.4, 30.7) | 52.3 (49.0, 55.0) | 79.1 (69.0, 85.0) | <0.001 |
Gender, male/female | 236/326 | 77/116 | 47/66 | 112/144 | 0.712 |
Race, White/Black/Asian/Other | 404/11/84/63 | 109/1/46/37 | 70/8/24/11 | 255/2/14/15 | <0.001 |
B cells | 4.32 (6.74, 9.85) | 8.09 (5.82, 11.35) | 7.21 (5.04, 9.69) | 5.72 (3.56, 8.74) | <0.001 |
CD16 neg monocytes | 6.23 (9.51, 13.63) | 8.03 (5.31, 12.10) | 8.15 (5.75, 11.38) | 11.34 (7.85, 15.87) | <0.001 |
CD16 pos monocytes | 0.28 (0.57, 1.04) | 0.40 (0.11, 0.94) | 0.47 (0.25, 0.73) | 0.74 (0.42, 1.33) | <0.001 |
CD4 T cells | 30.79 (23.87, 38.93) | 28.78 (23.21, 36.82) | 30.35 (24.32, 38.44) | 32.27 (24.58, 39.82) | 0..097 |
CD8 T cells | 12.69 (8.90, 18.22) | 16.13 (11.54, 20.42) | 13.27 (9.50, 18.63) | 10.74 (7.37, 15.35) | <0.001 |
Central Memory CD4 T cells | 9.02 (5.53, 13.76) | 6.21 (3.79, 10.05) | 9.27 (6.50, 13.76) | 11.41 (7.53, 16.56) | <0.001 |
Central Memory CD8 T cells | 1.71 (1.06, 2.69) | 1.34 (0.64, 2.15) | 1.87 (1.14, 3.06) | 1.81 (1.17, 2.89) | <0.001 |
Effector CD4 T cells | 1.86 (0.85, 3.38) | 2.66 (1.58, 4.38) | 1.93 (0.88, 3.30) | 1.38 (0.60, 2.65) | <0.001 |
Effector CD8 T cells | 2.08 (1.35, 3.36) | 1.92 (1.15, 3.33) | 2.25 (1.50, 3.54) | 2.11 (1.50, 3.34) | 0.084 |
Effector Memory CD4 T cells | 6.04 (4.54, 8.21) | 5.44 (3.91, 7.29) | 6.71 (4.83, 9.29) | 6.32 (4.83, 8.45) | <0.001 |
Effector Memory CD8 T cells | 2.08 (1.35, 3.36) | 1.92 (1.15, 3.33) | 2.25 (1.50, 3.54) | 2.11 (1.50, 3.34) | 0.036 |
Gamma Delta T cells | 4.44 (3.35, 6.46) | 4.76 (3.36, 7.42) | 4.31 (3.23, 5.88) | 4.39 (3.38, 6.02) | 0.155 |
Lymphocytes | 81.26 (76.67, 85.91) | 83.39 (78.39, 86.64) | 79.04, 87.42) | 79.42 (73.55, 83.71) | <0.001 |
Memory B cells | 0.68 (0.44, 1.15) | 0.87 (0.47, 1.25) | 0.72 (0.47, 1.15) | 0.56 (0.37, 0.99) | 0.004 |
Monocytes | 10.19 (6.92, 14.95) | 8.61 (5.87, 12.79) | 8.89 (6.16, 11.91) | 12.51 (8.47, 17.13) | <0.001 |
Naive B cells | 1.70 (0.96, 2.84) | 1.82 (1.23, 2.96) | 1.97 (1.05, 2.94) | 1.37 (0.70, 2.59) | <0.001 |
Naive CD4 T cells | 10.67 (6.16, 16.92) | 13.79 (8.85, 18.50) | 10.05 (6.27, 15.54) | 8.69 (4.71, 15.44) | <0.001 |
Naive CD8 T cells | 5.32 (3.21, 9.21) | 9.67 (6.66, 12.42) | 5.91 (3.79, 8.10) | 3.54 (2.18, 5.18) | <0.001 |
NK cells | 4.41 (2.46, 7.08) | 3.12 (1.55, 5.29) | 4.23 (2.62, 7.21) | 5.38 (3.05, 8.02) | <0.001 |
NKT cells | 4.54 (2.97, 6.69) | 4.25 (2.44, 6.59) | 4.55 (3.12, 6.69) | 4.78 (3.23, 6.81) | <0.001 |
Plasmablasts | 1.17 (0.77, 1.78) | 1.30 (0.83, 1.90) | 1.11 (0.81, 1.84) | 1.08 (0.76, 1.66) | 0.063 |
T cells | 51.65 (43.21, 59.21) | 54.75 (46.73, 59.93) | 51.12 (41.72, 59.46) | 49.70 (42.00, 57.29) | 0.001 |
Transitional B cells | 0.63 (0.43, 1.05) | 0.74 (0.48, 1.41) | 0.74 (0.44, 1.31) | 0.54 (0.34, 0.84) | <0.001 |
Tregs | 3.10 (2.17, 4.70) | 2.74 (1.96, 4.15) | 2.84 (2.06, 4.09) | 3.71 (2.44, 5.55) | <0.001 |
Data presented as median (P25, P75).
Table 2
Cytokines | All (n=952) | Young (n=344) | Middle-aged (n=132) | Elder (n=476) | P value |
---|---|---|---|---|---|
Age, years | 59.89 (29.19, 76.62) | 26.81 (23.02, 30.71) | 52.00 (48.42, 55.00) | 76.62 (68.49, 84.99) | <0.001 |
Gender, male/female | 406/546 | 155/189 | 55/77 | 196/280 | 0.525 |
Race, White/Black/Asian/Other | 738/20/106/88 | 220/7/61/56 | 83/10/26/13 | 435/20/106/88 | <0.001 |
BDNF | 3535.73 (244.86, 474.62) | 369.95 (295.18, 428.90) | 351.45 (213.40, 508.08) | 0.739 | |
CCL1 | 39.45 (27.30, 63.36) | 45.52 (22.41, 63.36) | 38.89 (29.48, 55.70) | 1.000 | |
CCL11 | 52.20 (37.55, 68.02) | 47.33 (33.31, 66.66) | 54.55 (40.61, 70.89) | 53.40 (39.37, 68.79) | 0.001 |
CCL13 | 29.66 (28.03, 31.29) | 28.03 (26.51, 28.60) | 30.72 (29.64, 31.33) | 0.242 | |
CCL17 | 12.11 (8.98, 15.23) | 8.98 (8.98, 9.98) | 15.23 (15.23, 15.23) | 0.317 | |
CCL2 | 65.19 (46.47, 92.62) | 64.23 (48.02, 90.23) | 59.75 (41.59, 93.47) | 67.69 (47.03, 94.80) | 0.161 |
CCL21 | 44.36 (28.55, 54.01) | 42.84 (27.11, 58.20) | 44.36 (29.77, 48.33) | 0.834 | |
CCL22 | 242.78 (194.88, 296.41) | 256.96 (195.33, 315.82) | 254.79 (235.28, 296.41) | 163.46 (109.72, 226.70) | 0.011 |
CCL24 | 136.74 (100.98, 164.41) | 110.16 (83.62, 170.76) | 140.72 (130.23, 155.79) | 0.345 | |
CCL27 | 34.36 (27.74, 39.30) | 45.48 (14.22, 54.28) | 33.38 (27.87, 37.10) | 0.439 | |
CCL3 | 59.78 (32.38, 121.61) | 63.38 (33.76, 116.09) | 54.54 (29.39, 104.99) | 58.22 (31.84, 147.49) | 0.450 |
CCL4 | 170.31 (74.88, 373.42) | 147.35 (58.87, 320.20) | 158.71 (69.54, 351.19) | 208.62 (89.54, 418.99) | <0.001 |
CCL5 | 1,185.13 (833.26, 1,579.79) | 1,242.71 (875.98, 1,659.50) | 1,140.99 (543.69, 1,591.10) | 1,122.02 (836.60, 1,539.60) | 0.025 |
CCL7 | 54.37 (37.83, 75.91) | 55.17 (37.20, 75.94) | 55.00 (38.39, 74.08) | 53.72 (37.87, 75.91) | 0.971 |
CCL8 | 40.76 (22.80, 48.05) | 41.52 (26.89, 62.63) | 33.21 (22.19, 47.26) | 0.345 | |
CD40LG | 263.58 (123.34, 825.38) | 358.14 (143.05, 893.76) | 167.75 (95.91, 645.53) | 241.19 (127.69, 823.92) | 0.002 |
CSF1 | 8.29 (4.19, 13.81) | 7.00 (4.45, 14.36) | 10.99 (4.16, 19.07) | 8.47 (3.39, 12.80) | 0.023 |
CSF2 | 101.07 (60.56, 161.15) | 105.06 (63.03, 164.06) | 106.96 (57.54, 153.83) | 95.96 (61.18, 164.15) | 0.548 |
CSF3 | 30.46 (16.30, 57.49) | 38.12 (22.45, 66.38) | 24.58 (14.14, 54.39) | 25.78 (15.95, 51.01) | <0.001 |
CX3CL1 | 118.61 (80.61, 188.60) | 141.19 (80.89, 191.78) | 83.45 (54.64, 112.25) | 114.29 (83.79, 164.45) | 0.311 |
CXCL1 | 34.12 (21.01, 61.64) | 39.93 (21.99, 66.53) | 28.92 (14.56, 60.77) | 31.02 (21.62, 59.38) | 0.036 |
CXCL10 | 85.80 (64.64, 111.72) | 83.21 (61.91, 110.49) | 84.68 (62.59, 118.26) | 87.22 (67.51, 111.87) | 0.396 |
CXCL12 | 74.47 (59.60, 112.61) | 82.86 (59.75, 119.99) | 73.18 (59.33, 111.78) | 0.684 | |
CXCL13 | 34.43 (27.24, 44.29) | 40.68 (27.24, 44.57) | 35.44 (26.67, 38.17) | 0.475 | |
CXCL5 | 104.31 (42.39, 209.94) | 129.24 (48.34, 273.15) | 90.25 (34.44, 206.45) | 94.11 (43.52, 184.36) | 0.017 |
CXCL8 | 30.02 (13.69, 72.21) | 22.32 (9.24, 38.77) | 33.68 (14.52, 120.40) | 40.95 (16.16, 93.53) | <0.001 |
CXCL9 | 115.97 (69.54, 193.35) | 85.41 (49.80, 128.23) | 102.97 (64.50, 170.43) | 150.65 (94.60, 243.76) | <0.001 |
EGF | 75.10 (51.09, 118.37) | 77.07 (51.93, 115.94) | 59.58 (30.49, 88.67) | 75.02 (35.14, 121.78) | 0.562 |
FASLG | 32.21 (20.41, 49.69) | 35.41 (22.30, 54.34) | 33.38 (19.20, 49.45) | 30.01 (19.83, 49.10) | 0.007 |
FGF2 | 21.99 (9.84, 43.82) | 25.08 (11.44, 43.98) | 15.64 (563, 43.19) | 22.35 (9.90, 43.98) | 0.003 |
FIGF | 24.58 (17.34, 33.38) | 27.25 (16.35, 41.72) | 24.32 (17.58, 32.61) | 0.429 | |
FLT3 | 29.31 (19.78, 37.79) | 31.24 (20.21, 41.38) | 28.18 (19.78, 34.34) | 0.600 | |
FLT3LG | 29.22 (19.12, 59.14) | 29.22 (19.68, 37.92) | 41.06 (12.00, 70.11) | 0.734 | |
HGF | 77.46 (56.39, 105.49) | 72.24 (51.02, 96.23) | 81.30 (53.62, 108.17) | 81.48 (58.82, 108.91) | 0.003 |
ICAM1 | 7,397.69 (3,437.47, 14,493.99) | 6,137.93 (3,159.17, 11,332.64) | 6,817.91 (2,305.43, 14,932.88) | 8,441.99 (4,250.30, 16,074.77) | 0.001 |
IFN1 | 5.24 (3.72, 12.42) | 5.10 (3.98, 8.18) | 3.86 (3.65, 11.85) | 5.41 (3.73, 13.68) | 0.003 |
IFNA2 | 55.97 (37.03, 82.82) | 59.77 (38.11, 85.73) | 41.40 (37.03, 45.77) | 51.15 (37.84, 61.07) | 0.395 |
IFNB1 | 9.70 (5.64, 14.65) | 10.51 (6.37, 19.51) | 9.70 (4.82, 14.60) | 9.01 (5.47, 13.78) | 0.013 |
IFNG | 27.49 (11.55, 48.80) | 28.46 (13.76, 56.66) | 30.63 (8.02, 56.48) | 26.77 (11.10, 43.83) | 0.034 |
IL10 | 10.79 (5.51, 20.52) | 8.23 (4.38, 18.33) | 15.01 (4.37, 35.82) | 12.23 (6.55, 19.73) | 0.001 |
IL12B | 36.83 (21.06, 55.41) | 32.94 (19.25, 55.36) | 40.32 (24.33, 53.26) | 37.38 (22.42, 56.24) | 0.330 |
IL13 | 31.71 (21.01, 44.06) | 34.57 (23.68, 48.12) | 32.44 (23.14, 44.25) | 29.21 (17.30, 42.07) | <0.001 |
IL15 | 44.18 (22.19, 73.01) | 49.73 (25.56, 84.08) | 46.61 (19.62, 84.47) | 41.14 (20.27, 65.28) | 0.005 |
IL16 | 57.44 (39.71, 81.02) | 81.02 (51.52, 86.65) | 47.48 (39.01, 57.44) | 0.059 | |
IL17A | 11.48 (5.26, 21.95) | 12.63 (5.76, 24.16) | 11.54 (4.38, 20.15) | 11.27 (5.50, 19.99) | 0.080 |
IL17F | 5.25 (3.96, 9.70) | 5.84 (4.25, 12.18) | 6.56 (3.98, 11.62) | 4.99 (3.74, 7.79) | <0.001 |
IL18 | 45.07 (29.82, 66.90) | 45.38 (22.92, 76.55) | 44.44 (29.82, 66.90) | 0.926 | |
IL1A | 32.00 (17.15, 52.19) | 32.57 (17.21, 51.14) | 32.98 (17.34, 57.12) | 31.46 (17.15, 51.97) | 0.810 |
IL1B | 9.57 (5.38, 17.58) | 10.51 (5.86, 18.78) | 10.01 (4.37, 17.72) | 8.95 (5.31, 15.47) | 0.519 |
IL1R1 | 257.35 (75.84, 956.86) | 146.93 (57.09, 996.39) | 269.09 (141.08, 1,156.05) | 305.58 (117.88, 931.47) | <0.001 |
IL2 | 71.01 (40.00, 127.03) | 82.54 (47.09, 135.86) | 59.42 (33.12, 144.48) | 66.10 (38.87, 114.32) | 0.005 |
IL20 | 58.35 (51.42, 60.79) | 60.23 (55.54, 60.86) | 58.03 (39.34, 58.35) | 0.327 | |
IL21 | 54.10 (35.23, 74.71) | 56.58 (56.09, 81.56) | 35.81 (35.14, 69.84) | 0.001 | |
IL22 | 70.57 (32.68, 110.09) | 79.54 (52.40, 127.96) | 68.02 (31.14, 109.91) | 0.559 | |
IL23A | 15.43 (15.12, 16.30) | 16.35 (16.07, 16.42) | 15.26 (15.07, 15.50) | <0.001 | |
IL27 | 17.77 (13.44, 65.71) | 13.96 (13.93, 54.42) | 24.26 (13.28, 68.53) | 0.575 | |
IL2RA | 80.02 (51.49, 93.44) | 80.49 (52.76, 94.13) | 51.67 (27.55, 75.78) | 0.240 | |
IL3 | 42.21 (35.26, 53.09) | 42.21 (35.26, 53.09) | |||
IL31 | 50.67 (50.55, 50.98) | 51.58 (46.62, 51.65) | 50.64 (50.59, 50.85) | 0.093 | |
IL4 | 20.76 (13.59, 29.39) | 19.10 (13.60, 30.64) | 20.92 (11.76, 30.42) | 20.84 (14.57, 28.14) | 0.575 |
IL5 | 105.79 (63.84, 175.78) | 129.32 (72.69, 207.79) | 103.35 (63.37, 164.80) | 95.86 (61.19, 152.80) | <0.001 |
IL6 | 16.95 (10.67, 39.13) | 13.19 (10.78, 24.34) | 22.18 (7.82, 160.10) | 20.45 (10.97, 52.57) | 0.014 |
IL7 | 151.26 (107.56, 214.44) | 166.74 (115.55, 243.33) | 136.40 (93.49, 218.07) | 145.12 (105.40, 197.04) | <0.001 |
IL9 | 45.82 (13.76, 46.42) | 14.29 (13.86, 33.43) | 46.28 (13.65, 46.50) | 0.168 | |
KITLG | 57.72 (32.57, 95.47) | 57.72 (32.54, 91.12) | 60.64 (33.09, 95.40) | 56.74 (32.42, 97.76) | 0.829 |
LEP | 1,598.66 (551.99, 3,541.07) | 1,425.99 (458.78, 3,307.60) | 1,309.77 (416.44, 3,077.36) | 1,736.76 (770.53, 3,664.17) | 0.012 |
LIF | 18.84 (12.92, 28.11) | 20.83 (13.09, 32.80) | 21.07 (14.27, 30.44) | 17.64 (12.56, 24.95) | <0.001 |
LTA | 6.30 (4.92, 10.53) | 6.21 (3.88, 10.89) | 7.07 (3.66, 12.61) | 6.33 (5.52, 9.44) | 0.136 |
NGF | 65.37 (39.60, 90.64) | 72.43 (42.49, 109.25) | 59.82 (36.40, 87.96) | 64.39 (39.76, 83.20) | 0.002 |
PIGF | 23.12 (11.16, 57.82) | 37.17 (11.33, 72.61) | 17.14 (11.13, 57.82) | 0.437 | |
RETN | 721.79 (394.87, 1,166.55) | 744.64 (426.01, 1,166.65) | 675.70 (246.63, 1,017.99) | 732.57 (392.27, 1,188.87) | 0.093 |
SERPINE1 | 1,737.60 (1,179.47, 2,690.94) | 1,994.88 (1,455.91, 2,916.09) | 1,404.73 (643.76, 1,835.73) | 1,697.76 (1,141.84, 2,608.45) | <0.001 |
TGFA | 17.45 (9.64, 27.21) | 16.98 (8.64, 27.14) | 20.32 (14.57, 31.78) | 16.97 (8.55, 24.85) | 0.024 |
TGFB1 | 19.76 (12.90, 29.66) | 21.02 (14.30, 33.99) | 18.61 (11.85, 30.52) | 18.85 (12.38, 26.68) | 0.002 |
TNF | 23.29 (11.48, 50.59) | 23.83 (11.56, 50.02) | 23.82 (12.01, 59.85) | 22.90 (11.48, 49.78) | 0.630 |
TNFSF10 | 73.59 (50.01, 105.06) | 77.87 (50.16, 107.92) | 70.27 (47.01, 100.03) | 71.70 (50.93, 105.68) | 0.661 |
VCAM1 | 3,160.31 (1,105.48, 6,676.43) | 4,044.48 (1,802.02, 7,496.88) | 3,136.24 (320.89, 5,695.18) | 2,795.18 (943.31, 6,574.75) | <0.001 |
VEGFA | 36.61 (21.32, 62.71) | 37.45 (22.34, 64.01) | 32.10 (18.75, 61.17) | 37.08 (21.33, 62.54) | 0.598 |
Data presented as median (P25, P75).
Table 3
Blood Count indices | All (n=1,155) | Young (n=244) | Middle-aged (n=369) | Elder (n=542) | P value |
---|---|---|---|---|---|
Age, years | 58.26 (48.00, 71.00) | 30.26 (25.72, 37.28) | 54.00 (50.04, 57.00) | 72.00 (65.51, 78.00) | <0.001 |
Gender, male/female | 431/724 | 101/143 | 250/331 | 211/331 | 0.041 |
Race, White/Black/Asian/Other | 928/42/103/82 | 192/19/10/23 | 262/16/55/36 | 474/7/38/23 | <0.001 |
Basophil count (K/μL) | 3.12 (3.09, 3.13) | 3.09 (3.04, 3.15) | 3.12 (3.10, 3.13) | 3.12 (3.11, 3.13) | <0.001 |
Basophil (%) | 0.39 (0.24, 0.67) | 0.39 (0.19, 0.67) | 0.40 (0.29, 0.69) | 0.39 (0.21, 0.59) | 0.076 |
Eosinophil count (K/μL) | 3.13 (3.07, 3.22) | 3.11 (3.02, 3.22) | 3.13 (3.08, 3.21) | 3.14 (3.08, 3.22) | 0.002 |
Eosinophil (%) | 2.17 (1.49, 2.95) | 2.20 (1.59, 2.71) | 2.17 (1.47, 3.11) | 2.10 (1.51, 2.97) | 0.938 |
HCT (%) | 43.25 (40.55, 45, 89) | 43.47 (40.50, 45.99) | 43.13 (40.49, 45.85) | 43.25 (40.69, 45.95) | 0.875 |
HGB (g/dL) | 14.71 (13.80, 15.70) | 14.81 (13.78, 16.01) | 14.70 (13.80, 15.60) | 14.70 (13.80, 15.60) | 0.230 |
Lymphocyte count (K/μL) | 3.81 (3.49, 4.21) | 3.92 (3.45, 4.46) | 3.84 (3.51, 4.21) | 3.75 (3.46, 4.12) | 0.013 |
Lymphocyte (%) | 31.76 (28.76, 34.66) | 31.87 (30.00, 33.57) | 32.06 (29.66, 35.96) | 31.45 (27.66, 34.26) | 0.023 |
MCH (pg) | 30.76 (29.62, 31.86) | 30.66 (29.44, 31.76) | 30.62 (29.52, 31.72) | 31.02 (29.82, 32.02) | 0.002 |
MCHC (g/dL) | 34.21 (33.71, 34.81) | 34.59 (34.00, 35.36) | 34.21 (33.71, 34.61) | 34.11 (33.61, 34.61) | <0.001 |
MCV (fL) | 90.11 (87.01, 92.86) | 88.79 (85.16, 91.00) | 89.91 (86.51, 92.11) | 91.11 (88.11, 93.31) | <0.001 |
Monocyte count (K/μL) | 3.34 (3.24, 3.45) | 3.30 (3.19, 3.49) | 3.33 (3.24, 3.42) | 3.36 (3.27, 3.46) | <0.001 |
Monocyte (%) | 8.27 (7.45, 9.23) | 8.31 (7.46, 9.06) | 8.25 (7.30, 9.10) | 8.26 (7.59, 9.35) | 0.246 |
Neutrophil count (K/μL) | 4.59 (3.89, 5.45) | 4.75 (3.79, 5.69) | 4.53 (3.86, 5.34) | 4.58 (3.94, 5.43) | 0.368 |
Neutrophil (%) | 56.48 (53.45, 60.25) | 56.26 (54.51, 58.68) | 56.48 (51.95, 60.65) | 56.55 (53.25, 60.65) | 0.754 |
Platelet count (K/μL) | 269.20 (236.20, 305.20) | 278.59 (241.00, 324.01) | 271.64 (241.20, 303.51) | 264.20 (231.20, 299.20) | 0.001 |
RBC (M/μL) | 4.96 (4.60, 5.26) | 4.88 (4.48, 5.21) | 5.00 (4.66, 5.28) | 4.97 (4.62, 5.27) | 0.026 |
RDW (%) | 12.39 (11.84, 13.19) | 12.10 (11.44, 13.03) | 12.44 (11.89, 13.12) | 12.49 (11.89, 13.29) | 0.003 |
WBC (K/μL) | 5.60 (4.66, 6.66) | 5.71 (4.63, 6.87) | 5.56 (4.66, 6.56) | 5.56 (4.76, 6.63) | 0.428 |
Data presented as median (P25, P75). HCT, hematocrit; HGB, hemoglobin; MCH, mean corpuscular hemoglobin; MCHC, mean corpuscular hemoglobin concentration; MCV, mean corpuscular volume; RBC, red blood cell; RDW, red blood cell distribution width; WBC, white blood cells.
Table 4
Blood lipid | All (n=664) | Young (n=30) | Middle-aged (n=250) | Elder (n=384) | P value |
---|---|---|---|---|---|
Age, years | 63.00 (54.00, 74.00) | 43.00 (42.00, 44.00) | 54.00 (50.00, 56.00) | 72.00 (66.50, 77.00) | <0.001 |
Gender, male/female | 239/425 | 9/21 | 72/178 | 158/226 | 0.005 |
Race, White/Black/Asian/Other | 507/10/99/48 | 16/0/9/5 | 164/8/54/24 | 327/2/36/19 | <0.001 |
Cholesterol/HDL (%) | 2.90 (2.40, 3.70) | 3.10 (2.20, 3.40) | 3.05 (2.50, 3.70) | 2.90 (2.40, 3.70) | 0.137 |
HDL cholesterol (mg/dL) | 64.00 (52.00, 79.00) | 60.50 (50.00, 73.00) | 64.00 (54.00, 79.00) | 63.00 (51.00, 79.00) | 0.409 |
LDL cholesterol (mg/dL) | 111.00 (89.00, 132.00) | 102.00 (83.00, 128.00) | 115.00 (96.00, 138.00) | 109.00 (86.00, 127.00) | 0.002 |
LDL/HDL (%) | 1.70 (1.30, 2.20) | 1.85 (1.20, 2.20) | 1.80 (1.40, 2.20) | 1.65 (1.25, 2.20) | 0.118 |
Non-HDL cholesterol Calc (mg/dL) |
128.00 (104.00, 149.00) | 118.00 (94.00, 145.00) | 132.50 (112.00, 154.00) | 124.00 (100.00, 146.00) | 0.004 |
Total cholesterol (mg/dL) | 195.00 (172.00, 218.00) | 177.50 (158.00, 201.00) | 199.00 (178.00, 225.00) | 192.00 (169.00, 216.00) | <0.001 |
Triglyceride (mg/dL) | 71.00 (50.00, 102.00) | 62.50 (47.00, 90.00) | 74.00 (52.00, 106.00) | 70.00 (50.00, 102.00) | 0.237 |
Data presented as median (P25, P75). HDL, high-density lipoprotein; LDL, low-density lipoprotein.
Table 5
Blood chemistry panel | All (n=664) | Young (n=30) | Middle-aged (n=250) | Elder (n=384) | P value |
---|---|---|---|---|---|
Age, years | 63.00 (54.00, 74.00) | 43.00 (42.00, 44.00) | 54.00 (50.00, 56.00) | 72.00 (66.50, 77.00) | <0.001 |
Gender, male/female | 239/425 | 9/21 | 72/178 | 158/226 | 0.005 |
Race, White/Black/Asian/Other | 507/10/99/48 | 16/0/9/5 | 164/8/54/24 | 327/2/36/19 | <0.001 |
Albumin total (g/dL) | 3.90 (3.70, 4.10) | 3.95 (3.80, 4.20) | 3.90 (3.70, 4.10) | 3.90 (3.70, 4.00) | 0.101 |
Alkaline phosphatase total (U/L) | 85.00 (72.50, 99.00) | 80.50 (61.00, 97.00) | 84.00 (70.00, 96.00) | 86.00 (74.50, 101.00) | 0.042 |
ALT SGPT (U/L) | 35.00 (31.00, 41.00) | 36.00 (32.00, 40.00) | 36.00 (31.00, 41.00) | 34.00 (31.00, 41.00) | 0.261 |
Anion gap (mmol/L) | 7.00 (6.00, 8.00) | 6.50 (5.00, 8.00) | 7.00 (5.00, 8.00) | 7.00 (6.00, 9.00) | 0.574 |
AST SGOT (U/L) | 22.00 (18.00, 26.00) | 20.50 (18.00, 23.00) | 21.50 (18.00, 26.00) | 22.00 (18.00, 26.00) | 0.400 |
C reactive protein (mg/dL) | 0.40 (0.30, 0.80) | 0.30 (0.20, 0.60) | 0.40 (0.30, 0.80) | 0.40 (0.30, 0.80) | 0.683 |
Calcium (mg/dL) | 9.00 (8.80, 9.30) | 9.00 (8.80, 9.20) | 9.10 (8.80, 9.30) | 9.00 (8.80, 9.30) | 0.641 |
Chloride (mmol/L) | 103.00 (101.00, 104.00) | 103.00 (102.00, 104.00) | 103.00 (101.00, 104.00) | 103.00 (101.00, 105.00) | 0.766 |
CO2 (mmol/L) | 29.00 (27.00, 30.00) | 29.00 (28.00, 30.00) | 29.00 (28.00, 31.00) | 29.00 (27.00, 30.00) | 0.390 |
Creatinine (mg/dL) | 0.90 (0.70, 1.00) | 0.80 (0.70, 0.90) | 0.85 (0.70, 1.00) | 0.90 (0.80, 1.00) | 0.012 |
ESR (mm/hr) | 8.50 (3.75, 17.50) | 4.00 (3.50, 15.00) | 9.00 (8.00, 20.00) | 0.514 | |
Globulin (g/dL) | 3.40 (3.20, 3.70) | 3.30 (3.10, 3.70) | 3.50 (3.20, 3.70) | 3.40 (3.20, 3.70) | 0.055 |
Glucose (mg/dL) | 86.00 (79.00, 93.00) | 81.50 (73.00, 89.00) | 86.00 (79.00, 92.00) | 86.00 (80.00, 94.00) | 0.039 |
Potassium (mmol/L) | 4.00 (3.80, 4.20) | 3.95 (3.70, 4.30) | 3.90 (3.70, 4.20) | 4.00 (3.80, 4.20) | 0.160 |
Protein total (g/dL) | 7.30 (7.10, 7.60) | 7.30 (7.00, 7.60) | 7.40 (7.20, 7.70) | 7.30 (7.00, 7.60) | 0.014 |
Sodium (mmol/L) | 139.00 (137.00, 140.00) | 139.00 (137.00, 140.00) | 139.00 (137.00, 140.00) | 139.00 (137.00, 140.00) | 0.988 |
Total bilirubin (mg/dL) | 0.60 (0.50, 0.80) | 0.60 (0.50, 0.60) | 0.60 (0.50, 0.80) | 0.60 (0.50, 0.80) | 0.324 |
Urea nitrogen (mg/dL) | 16.00 (13.00, 19.00) | 14.00 (13.00, 17.00) | 15.00 (13.00, 19.00) | 16.50 (14.00, 19.50) | 0.001 |
Data presented as median (P25, P75). ALT, alanine transaminase; SGPT, serum glutamic-pyruvic transaminase; AST, aspartate transaminase; SGOT, serum glutamic-oxaloacetic transaminase; ESR, erythrocyte sedimentation rate.
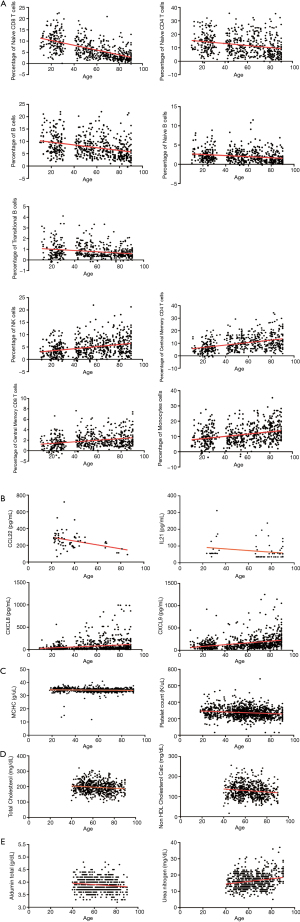
Correlation of indices to aging differed in genders
Coefficients of Spearman Rank Correlation were calculated between same index and aging in different genders, and the results indicated that some indices had different correlations to aging in different genders.
- In terms of cell population, Effector CD8 T cells and Effector Memory CD8 T cells showed positive correlations to aging in males, and Gamma Delta T cells and Plasmablasts showed negative correlations to aging in males. However, none of these showed correlations to aging in females. Meanwhile, CD4 T cells and Tregs showed positive correlations, and Memory B cells showed a negative correlation to aging only in females (Table 6, Figure 2A).
- In terms of cytokines, IFN1, LEP and RETN showed positive correlations to aging in males, and NGF, SERPINE1 and TGFB1 showed negative correlations to aging in males. Meanwhile, IL1R1 had a positive correlation to aging, while CXCL1, CXCL5, IL23A and FASLG showed negative correlations in females (Table 7, Figure 2B).
- In terms of complete blood count, neutrophil, red cell distribution width (RDW) and WBC showed positive correlations to aging, while HCT, HGB and RBC showed negative correlations to aging in males. Meanwhile, basophil and monocyte percentages showed positive correlations to aging, while lymphocyte count showed a negative correlation to aging in females (Table 8, Figure 2C).
- In terms of indices of blood lipids, Cholesterol to HDL ratio, low-density lipoprotein (LDL) cholesterol, LDL to HDL ratio, non-HDL cholesterol calculation, total cholesterol and Triglyceride showed negative correlations to aging in males (Table 9, Figure 2D).
- In terms of indices in blood chemistry panel, alanine transaminase (ALT) and serum glutamic-pyruvic transaminase (SGPT) showed negative correlations to aging in males. Meanwhile, glucose and urea nitrogen showed positive correlations to aging, and calcium and total protein showed negative correlations to aging in females (Table 10, Figure 2E).
Table 6
Cell Population | Female | Male |
---|---|---|
CD4 T cells | 0.144** | –0.043 |
Effector CD8 T cells | –0.029 | 0.164* |
Effector Memory CD8 T cells | 0.052 | 0.164* |
Gamma Delta T cells | –0.009 | –0.129* |
Memory B cells | –0.168** | –0.076 |
Plasmablasts | –0.004 | –0.229** |
Tregs | 0.323** | –0.074 |
*, P<0.05; **, P<0.01.

Table 7
Cytokines | Female | Male |
---|---|---|
CXCL1 | –0.119** | –0.032 |
CXCL5 | –0.185** | –0.029 |
FASLG | –0.133** | –0.098 |
TGFB1 | –0.004 | –0.145** |
SERPINE1 | –0.060 | –0.109* |
IFN1 | 0.040 | 0.115* |
IL1R1 | 0.141** | 0.069 |
LEP | 0.057 | 0.292** |
NGF | –0.046 | –0.102* |
RETN | 0.022 | 0.103* |
*, P<0.05; **, P<0.01.
Table 8
Blood count indices | Female | Male |
---|---|---|
HCT (%) | 0.041 | –0.164** |
RDW (%) | 0.060 | 0.144** |
Basophil count (K/μL) | 0.107** | 0.087 |
HGB (g/dL) | 0.011 | –0.239** |
Lymphocyte count (K/μL) | –0.101** | –0.093 |
Monocyte (%) | 0.160** | –0.025 |
Neutrophil Count (K/μL) | –0.029 | 0.120* |
RBC (M/μL) | 0.058 | –0.102* |
WBC (K/μL) | –0.052 | 0.104* |
*, P<0.05; **, P<0.01. HCT, hematocrit; volume; RDW, red blood cell distribution width; HGB, hemoglobin; RBC, red blood cell; WBC, white blood cells.
Table 9
Blood lipids | Female | Male |
---|---|---|
Cholesterol/HDL (%) | –0.038 | –0.249** |
LDL cholesterol (mg/dL) | –0.095 | –0.237** |
LDL/HDL (%) | –0.052 | –0.248** |
Non-HDL cholesterol Calc (mg/dL) | –0.076 | –0.242** |
Total cholesterol (mg/dL) | –0.062 | –0.169** |
Triglyceride (mg/dL) | 0.059 | –0.134* |
*, P<0.05; **, P<0.01. LDL, low-density lipoprotein; HDL, high-density lipoprotein.
Table 10
Blood chemistry panel indices | Female | Male |
---|---|---|
Calcium | 0.099* | –0.038 |
Urea nitrogen | 0.231** | 0.105 |
ALT SGPT | –0.048 | –0.0173** |
Glucose | 0.165** | 0.098 |
Protein total | –0.108* | –0.088 |
*, P<0.05; **, P<0.01. ALT, alanine transaminase; SGPT, serum glutamic-pyruvic transaminase.
Correlation of index to aging differed in races
Coefficients of Spearman Rank Correlation were calculated between the same index and age among different races, including Asian, Black, White and other races, and the results indicated that some indices had different correlations to aging among races.
- In terms of cell population, B cells, Central Memory CD4 T cells, Effector CD4 T cells, Naive CD4 T cells, Naive CD8 T cells and T cells showed correlations to aging in White, Asian and other races groups, but not in Black group. Among them, Central Memory CD4 T cells showed a positive correlation to aging, while other cells showed negative correlations. In White and other races groups, CD16+ monocytes and CD16− monocytes showed positive correlations to aging, while lymphocytes showed a negative correlation. CD8 T cells and Transitional B cells showed negative correlations in Asian and White. Meanwhile, Central Memory CD8 T cells, Effector Memory CD4 T cells, Effector Memory CD8 T cells, Naive B cells, NKT cells and Tregs showed correlations to aging only in White, among which Naive B cells showed a negative correlation while all others showed positive correlations. Furthermore, Memory B cells showed a negative correlation to aging in Asian and Black groups. NK cells showed a positive correlation to aging in Asian, Black and White, while Plasmablasts showed a negative correlation only in other races (Table 11, Figure 3A).
- In terms of cytokines, CCL11 and IL1R1 showed positive correlations, while FASLG and IL17F showed negative correlations to aging in Asian and White groups. CSF1, FGF2, IL12B, IL17A and TNFSF10 showed positive correlations only in Black, and CCL5 showed a negative correlation only in Asian. IL6 showed a positive correlation, while CSF3 and SERPINE1 showed negative correlations in Asian and other races groups. Furthermore, CXCL10, HGF, ICAM1, IFN1, IL4, LEP, LTA and VEGFA showed positive correlations, while CXCL5, IL13 and IL7 showed negative correlations only in White. CXCL9 and IL5 showed correlations to aging in White and other races, with CXCL9 being positive and IL5 being negative. IFNG and IL10 showed correlations in Asian and White, with IL10 being positive and IFNG being negative. IL1A and IL2 showed correlations in Black and White, with IL1A being positive in both groups. Interestingly, IL2 showed a positive correlation in Black, while showing a negative correlation in White. Lastly, IFNB1, LIF and NGF showed negative correlations in other races (Table 12, Figure 3B).
- In terms of Complete Blood Count test, basophil count showed a positive correlation to aging in both Black and White. eosinophil percentage and RBC showed positive correlations in Black. In White and other races, RDW percentage showed a positive correlation, while lymphocyte count showed a negative correlation. monocyte percentage showed a positive correlation in Black and other races. Some indices showed correlations only in White, in which eosinophil count, MCH, MCV and monocyte showed positive correlations, while HGB, lymphocyte percentage, MCHC and platelet count showed negative correlations (Table 13, Figure 3C).
- In terms of indices of blood lipids, cholesterol to HDL ratio showed a negative correlation only in Asian, while LDL cholesterol and non-HDL cholesterol Calc showed negative correlations only in White. Triglyceride showed a negative correlation only in Black. Furthermore, LDL to HDL ratio showed a negative correlation in Asian and White, while total cholesterol showed a negative correlation in White and other races (Table 14, Figure 3D).
- In terms of indices in blood chemistry panel, albumin total and calcium showed correlations to aging only in White, with albumin total being negative and calcium being positive. Meanwhile, creatinine, glucose and urea nitrogen showed positive correlations in Asian and White, while globulin showed a negative correlation only in Asian (Table 15, Figure 3E).
Table 11
Cell population | Asian | Black or African American | Other | White |
---|---|---|---|---|
B cells | –0.326** | 0.137 | –0.289* | –0.318** |
Monocytes | 0.127 | 0.000 | 0.263* | 0.304** |
CD8 T cells | –0.332** | –0.319 | –0.173 | –0.240** |
Central Memory CD8 T cells | 0.197 | 0.059 | 0.194 | 0.241** |
Central Memory CD4 T cells | 0.337** | 0.396 | 0.392** | 0.341** |
Effector CD4 T cells | –0.370** | –0.214 | –0.394** | –0.374** |
Naive CD4 T cells | –0.231* | 0.296 | –0.331** | –0.293** |
Naive CD8 T cells | –0.598** | –0.150 | –0.545** | –0.582** |
Tcells | –0.225* | 0.141 | –0.0261* | –0.187** |
Transitional B cells | –0.346** | –0.405 | –0.180 | –0.158** |
CD16 neg monocytes | 0.167 | 0.000 | 0.276* | 0.290** |
CD16 pos monocytes | 0.139 | 0.319 | 0.288* | 0.290** |
Effector Memory CD8 T cells | 0.008 | –0.018 | 0.189 | 0.175** |
Effector Memory CD4 T cells | 0.111 | –0.287 | 0.204 | 0.174** |
Lymphocytes | 0.004 | –0.005 | –0.311** | –0.295** |
Memory B cells | –0.399** | –0.770** | –0.175 | –0.044 |
NK cells | 0.326** | 0.733* | 0.141 | 0.325** |
Plasmablasts | –0.158 | 0.542 | –0.396** | –0.052 |
Naive B cells | –0.007 | 0.346 | –0.052 | –0.257** |
NKT cells | 0.128 | –0.146 | 0.216 | 0.177** |
Tregs | 0.169 | 0.096 | 0.109 | 0.118* |
*, P<0.05; **, P<0.01.

Table 12
Cytokines | Asian | Black or African American | Other | White |
---|---|---|---|---|
CCL5 | –0.269** | –0.307 | –0.013 | –0.014 |
CSF3 | –0.239* | 0.155 | –0.286** | –0.063 |
CXCL10 | 0.147 | –0.245 | 0.151 | 0.155** |
CXCL5 | –0.066 | –0.363 | –0.205 | –0.088* |
CXCL9 | 0.154 | 0.449 | 0.437** | 0.461** |
FASLG | –0.300** | 0.349 | 0.149 | –0.115** |
FGF2 | 0.033 | 0.583* | 0.016 | 0.064 |
HGF | –0.068 | 0.165 | –0.099 | 0.153** |
ICAM1 | –0.109 | –0.319 | –0.063 | 0.221** |
IFN1 | 0.014 | 0.633 | 0.095 | 0.095* |
IFNB1 | 0.019 | 0.080 | –0.266* | –0.004 |
IFNG | –0.272** | 0.617* | –0.181 | –0.095* |
IL10 | 0.344** | 0.615* | 0.174 | 0.144** |
IL13 | –0.095 | –0.035 | –0.208 | –0.109** |
IL17F | –0.266* | –0.437 | –0.207 | –0.159** |
IL1R1 | 0.246* | 0.210 | 0.026 | 0.105** |
IL4 | 0.083 | 0.156 | –0.087 | 0.145** |
IL5 | –0.133 | 0.248 | –0.344** | –0.102** |
IL6 | 0.420** | 0.600 | 0.477** | 0.023 |
IL7 | 0.071 | 0.202 | –0.200 | –0.105** |
LEP | –0.147 | –0.451 | –0.006 | 0.199** |
LIF | 0.078 | 0.019 | –0.251* | –0.069 |
LTA | 0.100 | –0.055 | –0.052 | 0.175** |
NGF | 0.089 | –0.080 | –0.263* | –0.013 |
*, P<0.05; **, P<0.01.
Table 13
Blood Count indices | Asian | Black or African American | Other | White |
---|---|---|---|---|
MCH | 0.193 | 0.027 | 0.002 | 0.092** |
MCHC | 0.103 | –0.097 | –0.042 | –0.0188** |
Basophil count | 0.016 | 0.663** | –0.203 | 0.100** |
RDW percent | 0.098 | –0.133 | 0.275* | 0.113** |
Eosinophil count | –0.002 | 0.304 | –0.035 | 0.109** |
Eosinophil percent | –0.065 | 0.472** | 0.093 | 0.016 |
Monocyte count | 0.140 | –0.077 | –0.045 | 0.162** |
Monocyte percent | 0.047 | 0.314* | 0.328** | 0.028 |
RBC | –0.046 | 0.316* | –0.065 | 0.001 |
HGB | 0.056 | 0.173 | –0.172 | –0.105** |
Lymphocyte count | –0.024 | –0.083 | –0.288** | –0.083* |
MCV | 0.148 | –0.028 | 0.076 | 0.213** |
Platelet count | 0.010 | –0.119 | –0.043 | –0.145** |
*, P<0.05; **, P<0.01. MCH, mean corpuscular hemoglobin; MCHC, mean corpuscular hemoglobin concentration; RDW, red blood cell distribution width; RBC, red blood cell; HGB, hemoglobin; MCV, mean corpuscular volume.
Table 14
Blood Lipids | Asian | Black or African American | Other | White |
---|---|---|---|---|
Cholesterol/HDL (%) | –0.207* | –0.292 | –0.068 | –0.067 |
LDL/HDL (%) | –0.202* | –0.272 | –0.091 | –0.094* |
Total Cholesterol (mg/dL) | –0.055 | –0.146 | –0.293* | –0.101* |
Triglyceride (mg/dL) | –0.153 | –0.736* | –0.079 | 0.071 |
LDL cholesterol (mg/dL) | –0.102 | 0.079 | –0.193 | –0.153** |
Non-HDL cholesterol Calc (mg/dL) | –0.145 | –0.158 | –0.235 | –0.116** |
*, P<0.05; **, P<0.01. LDL, low-density lipoprotein; HDL, high-density lipoprotein.
Table 15
Blood chemistry penal indices | Asian | Black or African American | Other | White |
---|---|---|---|---|
Globulin | –0.216* | –0.076 | 0.281 | 0.029 |
Glucose | 0.264** | –0.018 | 0.077 | 0.167** |
Urea nitrogen | 0.211* | –0.142 | 0.060 | 0.169** |
Creatinine | 0.225* | –0.222 | –0.057 | 0.192** |
Albumin total | –0.028 | 0.327 | –0.178 | –0.130** |
Calcium | 0.054 | –0.126 | –0.107 | 0.097* |
*, P<0.05; **, P<0.01.
Screening of crucial genes closely related to aging and biological functions of DEGs enrichment
To screen potential biomarkers related to aging, gene expression data were analyzed. Samples were divided into two groups based on age, with >60 being Old group, and <60 being Young group. There were totally 101 differential genes identified. Compared to the Young group, 63 genes were up-regulated and 38 genes were down-regulated in the Old group (Figure 4A), and a heat map was constructed (Figure 4B). We then performed GO enrichment analysis by using DAVID (https://david.ncifcrf.gov/) with keywords: immune response, cell surface receptor, signaling pathway, cell chemotaxis, B cell proliferation and platelet degranulation (Figure 4C).
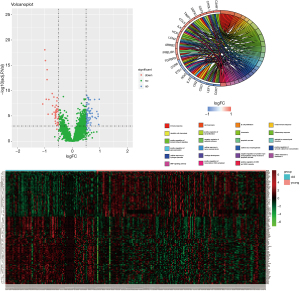
Protein-Protein Interaction Network analysis
The protein-protein interaction network of DEGs was constructed by using STRING database, and 61 proteins were shown associated (Figure 5). A protein interaction network of differential gene expression is shown in Figure 5A, and the number of core gene nodes selected from the interaction network is shown in Figure 5B, with the node number of 61 genes being ranked from small to large. Genes within the top 10% and enriched in the first five functions were selected, including CCL5, SERPINA1, MS4A1, ORM2, ETS1, CD27 and IL7R.
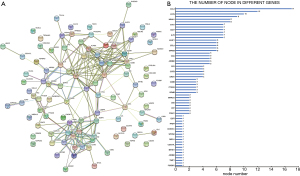
Discussion
Aging and its related immunodeficiency are associated with increased systemic inflammation, and are also main risk factors for many diseases, including neurodegenerative diseases, cardiovascular diseases and tumors. The biomarkers for the aging of immune system are crucial to evaluate host immune status and develop optimal immunotherapeutic regimens. In this study, we explored the correlation between aging and various indices in cell population profiling, Complete Blood Count and blood chemistry panel, in an attempt to identify features which were associated with the aging of immune system and could be used as potential biomarkers.
Our analysis indicated that NK cell number had a positive correlation with aging, which is consistent with a previous study (10). Notably, increase in NK cell number plays a role in the vicious circle of chronic inflammation, which promotes the geriatric disease atherosclerosis (11), and associated with premature biological aging (12). Meanwhile, monocytes increase along aging. Monocytes are a central driving factor for vascular inflammation in atherosclerosis, involving in the development of the disease, from the earliest asymptomatic atherosclerotic lesions to the final rupture of plaque, resulting in potentially fatal consequences (13). It’s also showed that the number of B lymphocytes, including B cells, Naive B cells and transitional B cells, had a negative correlation with aging. B lymphocytes play a pivotal role in humoral immunity and, as powerful APC, are crucial for the development of memory T cells. It’s reported that the B cell versatility showed dramatic decrease in some very aged individuals, which was a strong indicator for poor physical condition in the elderly (9). Transitional B cells play an important role in the negative selection of lymphocyte development and the progression of autoimmune diseases (14), thus its reduction could affect the humoral immunity in the aged. In addition, Memory T cells showed a positive correlation, while Naive T cells showed a negative correlation to aging (15). Our study demonstrated that immune cell populations were greatly affected with aging, and Transitional B cells could be used as a biomarker for aging.
By analyzing the changes of blood cytokines in different age groups, we found several cytokines related to aging, including CXCL9, CCL22, IL21, IL23A and IL31. In this study, CXCL9 was the only one showing a positive correlation to aging, while others showed negative correlations. CXCL9 affects the growth, movement, or activation state of the cells, and participates in immune and inflammatory responses. It’s chemotactic for activated T cells through binding to CXCR3. It’s reported that increased CXCL9 was associated with falls, which might help to correctly understand the mechanism of falls in the elderly (16). CCL22 may play a role in the trafficking of activated/effector T cells to inflammatory sites and other physiological aspects of activated T cells. It’s a mild chemoattractant for primary activated T cells and a potent chemoattractant for chronically activated T cells through binding to CCR4 receptor. However, it has no chemoattractant activity for neutrophils, eosinophils, or resting T cells. IL21 is a cytokine with immunoregulatory activity, promoting the transition between innate and adaptive immunity. It has been proved that IL21 could enhance the thymus production in aged mice (17). IL23A associates with IL12B to form the IL-23 interleukin, a heterodimeric cytokine which functions in innate and adaptive immunity. IL31 associates with OSMR to form the interleukin-31 receptor, which activates STAT3 and STAT1/STAT5 to a lower extent. The relationship between IL23A/IL31 and aging has not been reported in the literature, and our results suggest that they are worthy of further study, and might be used as new biomarkers for aging.
The results of functional gene enrichment and protein interaction analysis showed that differential genes were enriched in pathways related to B cell proliferation and other immuno-signal transduction, indicating that these genes might be highly interacted and play a core role in the process of biological aging. The expression levels of CCL5, SERPINA1 and ORM2 were increased in the aged groups. CCL5 is the chemotaxis of memory T helper cells and eosinophils, and can cause basophils to release histamine and activate eosinophils. SERPINA1 may be associated with Parkinson's disease (18). ORM2 acts as a transport protein in the blood and seems to play a role in regulating the activity of the immune system during the acute response. Meanwhile, the expression levels of MS4A1, ETS1, CD27 and IL7R genes decreased with aging. MS4A1 is a B-lymphocyte-specific membrane protein that plays a role in the regulation of cellular calcium influx necessary for the development, differentiation, and activation of B-lymphocytes. ETS1 can promote apoptosis of endothelial cells (19) and plays a role in maintaining the proliferation of cardiomyocytes. It has been found that the expression of ETS1 is significantly decreased in aging cardiomyocytes (20). CD27 stimulates T cell proliferation and is an initial cell marker (21). It’s also down-regulated in the elderly. IL7R has been reported to be lower in the elderly, and some believe that the decreased IL7R may be related to longevity, and the higher expression of IL7R gene is associated with immune-related diseases (22). It has also been reported that IL7R plays a role in age-related neurodegenerative diseases (23). Although its role in aging has not yet been clearly defined, there is no denying that the expression of IL7R does change with aging. Taken together, SERPINA1, ORM2, MS4A1, ETS1, CD27 and IL7R might be used as potential biomarkers of aging.
Analysis on indices in Complete Blood Count test showed that Basophil, MCH and MCV were positively correlated with aging, while MCHC and Platelet were negatively correlated with aging. Meanwhile, blood UREA NITROGEN, CREATININE and GLUCOSE were positively correlated with aging, while ALBUMIN and PROTEIN were negatively correlated with aging. Furthermore, blood cholesterol/HDL ratio, LDL cholesterol, LDL/HDL ratio, non-HDL cholesterol Calc, total cholesterol and Triglyceride were negatively correlated with aging. These are useful indicators for many diseases, however their relationship to age-related diseases is still not clear. Further work will be needed to investigate their potential as biomarkers for aging.
It’s interesting that some correlations seemed exclusive to certain genders or races. For example, the significant association between blood lipids and aging was observed only in males. It is well known that abnormalities in blood lipids are risk factors for some diseases. Does this indicate the prevalence of certain diseases in the male population, and is it associated with aging? In addition, we also found that CCL5, Cholesterol/HDL (%) and globulin were significantly associated with aging only in Asian population, and there was no difference among other races group. All these indicate that the changes in the process of aging are affected by multiple factors and more work is needed to elucidate the underlying mechanisms.
Acknowledgments
We would like to thank Dr. Oscar Junhong Luo for his help in polishing our paper.
Funding: This work was supported by National Natural Science Foundation of China [No. 31741037 to G. C.], Natural Science Foundation of Guangdong Province [No. 2014A030313370 to P. W.] and Medical Scientific Research Foundation of Guangdong Province [No. A2017386 to P. W.].
Footnote
Reporting Checklist: The authors have completed the MDAR reporting checklist. Available at https://aob.amegroups.com/article/view/10.21037/aob-20-79/rc
Peer Review File: Available at https://aob.amegroups.com/article/view/10.21037/aob-20-79/prf
Conflicts of Interest: All authors have completed the ICMJE uniform disclosure form (available at https://aob.amegroups.com/article/view/10.21037/aob-20-79/coif). The authors have no conflict of interest to declare.
Ethical Statement: The authors are accountable for all aspects of the work in ensuring that questions related to the accuracy or integrity of any part of the work are appropriately investigated and resolved. The study was conducted in accordance with the Declaration of Helsinki (as revised in 2013).
Open Access Statement: This is an Open Access article distributed in accordance with the Creative Commons Attribution-NonCommercial-NoDerivs 4.0 International License (CC BY-NC-ND 4.0), which permits the non-commercial replication and distribution of the article with the strict proviso that no changes or edits are made and the original work is properly cited (including links to both the formal publication through the relevant DOI and the license). See: https://creativecommons.org/licenses/by-nc-nd/4.0/.
References
- Bratic A, Larsson NG. The role of mitochondria in aging. J Clin Invest 2013;123:951-7. [Crossref] [PubMed]
- Putin E, Mamoshina P, Aliper A, et al. Deep biomarkers of human aging: Application of deep neural networks to biomarker development. Aging (Albany NY) 2016;8:1021-33. [Crossref] [PubMed]
- Mamoshina P, Volosnikova M, Ozerov IV, et al. Machine Learning on Human Muscle Transcriptomic Data for Biomarker Discovery and Tissue-Specific Drug Target Identification. Front Genet 2018;9:242. [Crossref] [PubMed]
- Peters MJ, Joehanes R, Pilling LC, et al. The transcriptional landscape of age in human peripheral blood. Nat Commun 2015;6:8570. [Crossref] [PubMed]
- Wagner KH, Cameron-Smith D, Wessner B, et al. Biomarkers of Aging: From Function to Molecular Biology. Nutrients 2016;8:338. [Crossref] [PubMed]
- Arai Y, Martin-Ruiz CM, Takayama M, et al. Inflammation, But Not Telomere Length, Predicts Successful Ageing at Extreme Old Age: A Longitudinal Study of Semi-supercentenarians. EBioMedicine 2015;2:1549-58. [Crossref] [PubMed]
- Zierer J, Menni C, Kastenmüller G, et al. Integration of 'omics' data in aging research: from biomarkers to systems biology. Aging Cell 2015;14:933-44. [Crossref] [PubMed]
- McElhaney JE, Effros RB. Immunosenescence: what does it mean to health outcomes in older adults? Curr Opin Immunol 2009;21:418-24. [Crossref] [PubMed]
- Qin L, Jing X, Qiu Z, et al. Aging of immune system: Immune signature from peripheral blood lymphocyte subsets in 1068 healthy adults. Aging (Albany NY) 2016;8:848-59. [Crossref] [PubMed]
- Müller L, Di Benedetto S, Pawelec G, et al. The Immune System and Its Dysregulation with Aging. Subcell Biochem 2019;91:21-43. [Crossref] [PubMed]
- Bonaccorsi I, Spinelli D, Cantoni C, et al. Symptomatic Carotid Atherosclerotic Plaques Are Associated With Increased Infiltration of Natural Killer (NK) Cells and Higher Serum Levels of NK Activating Receptor Ligands. Front Immunol 2019;10:1503. [Crossref] [PubMed]
- Wang JC, Bennett M, et al. Aging and atherosclerosis: mechanisms, functional consequences, and potential therapeutics for cellular senescence. Circ Res 2012;111:245-59. [Crossref] [PubMed]
- Rogacev KS, Cremers B, Zawada AM, et al. CD14++CD16+ monocytes independently predict cardiovascular events: a cohort study of 951 patients referred for elective coronary angiography. J Am Coll Cardiol 2012;60:1512-20. [Crossref] [PubMed]
- Ikemiyagi M, Hirai T, Ishii R, et al. Transitional B Cells Predominantly Reconstituted After a Desensitization Therapy Using Rituximab Before Kidney Transplantation. Ther Apher Dial 2017;21:139-49. [Crossref] [PubMed]
- Ferrando-Martínez S, Ruiz-Mateos E, Hernández A, et al. Age-related deregulation of naive T cell homeostasis in elderly humans. Age (Dordr) 2011;33:197-207. [Crossref] [PubMed]
- Amorim JSC, Torres KCL, Teixeira-Carvalho A, et al. Inflammatory markers and occurrence of falls: Bambuí Cohort Study of Aging. Rev Saude Publica 2019;53:35. [Crossref] [PubMed]
- Al-Chami E, Tormo A, Pasquin S, et al. Interleukin-21 administration to aged mice rejuvenates their peripheral T-cell pool by triggering de novo thymopoiesis. Aging Cell 2016;15:349-60. [Crossref] [PubMed]
- Siitonen A, Nalls MA, Hernández D, et al. Genetics of early-onset Parkinson's disease in Finland: exome sequencing and genome-wide association study. Neurobiol Aging 2017;53:195.e7-195.e10. [Crossref] [PubMed]
- Sato Y, Teruyama K, Nakano T, et al. Role of transcription factors in angiogenesis: Ets-1 promotes angiogenesis as well as endothelial apoptosis. Ann N Y Acad Sci 2001;947:117-23. [Crossref] [PubMed]
- Ball AJ, Levine F. Telomere-independent cellular senescence in human fetal cardiomyocytes. Aging Cell 2005;4:21-30. [Crossref] [PubMed]
- Cao JN, Gollapudi S, Sharman EH, et al. Age-related alterations of gene expression patterns in human CD8+ T cells. Aging Cell 2010;9:19-31. [Crossref] [PubMed]
- Passtoors WM, van den Akker EB, Deelen J, et al. IL7R gene expression network associates with human healthy ageing. Immun Ageing 2015;12:21. [Crossref] [PubMed]
- Brinkmeyer-Langford CL, Guan J, Ji G, et al. Aging Shapes the Population-Mean and -Dispersion of Gene Expression in Human Brains. Front Aging Neurosci 2016;8:183. [Crossref] [PubMed]
Cite this article as: Sun M, He X, Mao L, Ma T, Deng J, Gao L, Wang P, Chen G. Correlation analyses between age and indices in routine blood laboratory tests suggest potential aging biomarkers. Ann Blood 2022;7:36.