Recent advances in blood group genotyping
Introduction
Red blood cell (RBC) antigen typing of both recipients and donors is vital for safe and effective transfusion. Despite the existence of >330 RBC antigens, current routine pre-transfusion testing only includes matching the patient and donor for ABO and D using methods that have not materially changed for over 60 years despite clinically significant limitations. Approximately 3% of Caucasian recipients and 30–50% of patients of African ancestry receiving chronic transfusion therapy will form an anti-RBC antigen alloantibody (1-3). Once a patient is sensitized, they are at increased risk of developing additional alloantibodies, and must receive donor units tested and found to be negative for those antigens to avoid transfusion reactions (4). However, alloantibody complications following transfusion has been an accepted level of risk in the absence of efficient, affordable, and scalable extended antigen typing strategy.
Traditional antibody-based serologic typing methods are labor intensive and difficult to scale for routine non-ABO/D antigen typing (i.e., extended antigen typing). In addition, serologic reagents are not commercially available for all clinically significant antigens (e.g., U, Doa, Dob, Jra, Lan, Vel). These limitations, especially reagent availability, can be addressed with DNA-based genotyping by using genetic information to predict the RBC antigen phenotype. The current genotyping assays on the market largely rely on PCR amplification to detect single nucleotide variants (SNVs) either directly (e.g., allele specific primers) or by secondary detection (e.g., probe hybridization or Sanger sequencing) (5). While PCR-based genotyping can be used to broadly genotype most antigens, current assay formats limit the number of SNVs and samples that can be simultaneously tested (~50 SNVs and ~100 samples per run). In addition, PCR-based assays detect structural variations (SVs) with PCR primers spanning the breakpoint region, which requires that the breakpoints are well characterized and many are not. This means that current PCR-based genotyping assays are not able to fully characterize all genetically understood antigens, especially the highly polymorphic blood group systems such as ABO, Rh, and MNS. In addition, PCR-based genotyping assays often represent a significant cost and time investment per sample and thus can only be performed on a subset of all blood donors.
The emergence of RBC antigen genotyping using next-generation sequencing (NGS) and high-density DNA arrays has the potential to overcome many of the above limitations. However, to fully realize this potential many technical challenges needed to be overcome: (I) lack of array and NGS data with paired conventional serology or PCR-based results; (II) need for fully annotated and complete electronic databases of RBC antigen allele genotypes to phenotypes; (III) published RBC genetic changes were defined using cDNA sequences without mapping to human reference genome coordinates; and (IV) lack of software capable of automatically interpreting the large amount of data. Over the last decade many groups have addressed these challenges (Figure 1) (6-28).
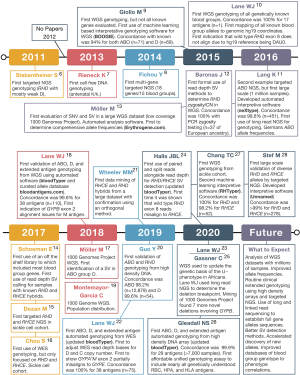
Despite the above technical advancements in NGS and high-density DNA array genotyping, its clinical adoption will require addressing the following implementation challenges: (I) turnaround time is adequate and/or results used at next donation, (II) scalable assay capable of genotyping large numbers of donors, and (III) affordable so it does not drastically increase the cost of blood unit acquisition.
Speed vs. size vs. cost
Oftentimes different testing methodologies are a tradeoff between speed and accuracy (Figure 2A). For example, D serologic antigen typing can be performed within an hour at low cost. However, PCR genotyping can determine the weak and partial D status costs and can take between hours to several days depending on the specific assay and genetic changes present. As a result, the scalability or the number of samples and different antigens that can be tested by serology at one time is low, but this can be improved by PCR genotyping at a higher cost (Figure 2B). As such every transfusion recipient and donor undergoes D serologic testing, but only a select few undergo RHD PCR genotyping.
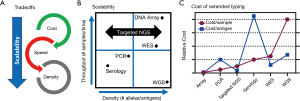
In order to achieve a future in which the full antigen results are known for every blood donor there is a need for new technologies that break these tradeoffs; like NGS and high-density DNA arrays. For example, scalability is built directly into NGS which has the ability to characterize all genetically understood antigens using whole-genome sequencing (WGS) or it can be scaled to whole-exome sequencing (WES), specific blood groups, or even just a single SNV (Figure 2B). High-density DNA arrays have seen a resurgence due to large genome-wide association studies (GWAS) (29). DNA arrays are capable of scaling to detect all known genetic blood group changes at a cost much more favorable than even targeted NGS (Figure 2C) (26).
Although comprehensive, it takes several days to perform genotyping with targeted NGS and high-density DNA arrays and thus they are best suited for particular clinical situations including: (I) samples that do not require immediate results (e.g., stem cell donor screening), (II) cases that have eluded other methods that would benefit from the comprehensive look (e.g., unresolved alloantibody workups), and (III) genotyping of repeat blood donors where the results can be used for future donations. Although comprehensive genotyping of large blood donor cohorts opens up the door for routine extended matching, it will also require the development of product selection algorithms that prioritize alloantibody prevention without putting undo strains on blood product inventory. For a detailed discussion of the clinical use of genotyping in transfusion medicine readers are referred to the recent review on this topic by Westhoff (5).
WGS
WGS is the most comprehensive form of NGS, since all genomic DNA is sequenced. Since DNA is not enriched during WGS it has the most even sequence coverage of any NGS technique, which offers the best opportunity for read depth of coverage copy number (CN) analysis to detect SVs. To date, WGS based genotyping has either been on existing large datasets with no or limited concordance testing (~1,000–3,000 samples) or smaller datasets with concordance testing (~20–200 samples). In many cases genotyping and concordance was done alongside ongoing genomics projects in individuals already undergoing WGS including: MedSeq Project (10,12,18,22,23,27,30), MilSeq Project (22), Sickle Cell Clinical Research and Intervention Program (SCCRIP) (27), and Jackson Heart Study (21).
In 2015, Giollo et al. (9) was first to develop automated interpretative software for genotyping many, but not all known blood group genes, along with a concordance of 94% for ABO (n=71) and D (n=69). In 2016, Lane et al. (10) was the first to publish WGS-based genotyping for all known blood group genes by manual analysis of WGS data from one individual with a concordance of 100% for 17 antigens. This was followed in 2016 by Möller et al. (13), who developed Erythrogene and analyzed 1000 Genomes Project WGS to determine population frequencies for blood group antigen alleles but without any concordance testing. In 2018, Lane et al. (18) published on the development of automated interpretive software (bloodTyper) capable of genotyping all known blood group genes, with a 99.8% concordance for 38 antigens (n=110). Subsequent WGS papers either focused on samples with complex or uncommon changes, especially SVs, in the ABO (17), Rh (21,24,27), and MNS (23,25,31) blood group systems. In particular, a range of SVs from small (e.g., RHCE*CeRN with ~500 bp conversion) to large [e.g., RHD*DIIIa-CE(4-7)-D with ~16,000 bp conversion] can be correctly assigned from WGS data even with automated interpretive software (21,24,27).
WGS is a particularly powerful tool for the unbiased evaluation of genetic changes. For example, WGS of known U– phenotype samples showed that the genetic basis for U– in those of African Ancestry was actually the result of two different full gene deletions of GYPB, with seven other novel deletions with U– potential identified in the 1000 Genomes Project WGS data (23,25). Additionally, WGS has been used to determine the geographic distribution of blood group alleles (19) and to better understand the genetic basis of blood group systems controlled by genetic changes in transcription factor binding regions (e.g., P1 and Xga) (30).
It is important to note that much of the above analysis was only possible due to the development of fully automated interpretive software developed by several different groups including: BOOGIE (9), Erythrogene (13), bloodTyper (18,22,24), and RHTyper (27). Furthermore, Möller et al. (13) and Lane et al. (18) developed their own curated blood group allele databases http://erythrogene.com and http://bloodantigens.com, respectively. In addition, the vast majority of the other projects utilized semi-automated pipelines to enable their analysis (19,21,23).
Figure 3 shows a representative NGS workflow indicating how SNVs, indels, and SVs can be determined from the underlying data to determine the final genotype. In particular, the ability to cis/trans-phase nearby heterozygous genetic changes using the clonal NGS reads offers a major advantage over Sanger sequencing (Figure 3). In addition, the ability to perform CN analysis using different methods such as read depth, paired reads, and split reads offers a powerful generalized toolbox for detecting SVs including deletions, gene conversions, and recombinations (23,24). Read depth is a measure of the number of reads across a given region which can be compared to a stable 2× copy control region, to determine the CN of the given region (e.g., the ratio of reads over RHD to a control region will yield the RHD CN) (12). In general, the fragments of DNA sequenced are a few 100 bp long, so when the forward and reverse paired reads align further than the expected distance it is likely due to the DNA fragment spanning across a breakpoint (e.g., across a large deletion or between two genes when a hybrid is present). It should be noted that when breakpoints occur in very large regions of high identity longer than the DNA fragment sizes (e.g., 1,000 bp) then it is not possible for paired reads to span the breakpoint (23). Split reads occur in special circumstances when the breakpoint region is very small or an indel occurs at the breakpoint. As such, when split reads are present either the forward or reverse read will partially contain DNA from a region that is not normally adjacent (e.g., 109 bp delins present in RHCE*C) (24). When a deletion is present there will be an absence of reads (low read depth) and paired reads spanning the region missing reads (23). When a hybrid is present, there will be absence of reads over the region replaced in the hybrid gene with an increase in the region that replaces, along with paired reads between the two gene regions (24). Figure 3 shows an example of how WGS can be used to call RHD zygosity using read depth and how the RHCE*C exon 2 gene conversion CE-D(2)-CE can be called using a combination of read depth, paired reads, and split reads.
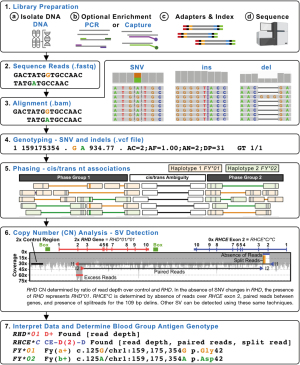
Targeted NGS
One of the major benefits of targeted NGS is that sequencing of only enriched regions allows the cost of the flow cell to be spread across more samples. As such targeted NGS can scale for each use case from a just single SNV (7) to essentially all RBC antigen genes (22). Enrichment of DNA for targeted NGS can be accomplished by several different methods including: long-range PCR, amplicon enrichment using many overlapping small PCR reactions, and hybrid capture using bead-conjugated DNA probes to pulldown desired regions after fragmentation. WES is a special case of targeted NGS in which exons are enriched genome wide often using off the shelf commercially available kits. WES has been shown to enrich for nearly all known blood group changes including those in nearby intronic regions, with the exception of non-coding regions required for P1, Xga, and a few rare ABO subtypes (22). WES also represents a cost effective way to discover new blood group genes and has been used to define the following blood group systems: Vel (32), KANNO (33), CTL2 (34), PEL (35), and MAM (36). In addition, commercial kits are available that enrich for only clinical relevant genes through the genome, which has been shown to contain many relevant RBC antigen genes (14). However, the cost of WES and clinical exome enrichment is higher than blood group focused enrichment panels (Figure 2C).
In 2011, Stabentheiner et al. (6) was the first to publish a NGS-based genotyping study of any kind, which included targeted RHD NGS focused on SNV based alleles. In 2014, Fichou et al. (8) published the first targeted NGS panel to enrich for multiple RBC antigen genes with 18 genes in 15 systems, but they required the addition of allele-specific PCR reactions to sequence GYPA*01/*02 and RHCE*C/*c. This difficulty performing NGS on the Rh and MNS blood group systems highlights the challenges that have since been solved with targeting, sequencing, and aligning homologous genes (e.g., RHD/RHCE and GYPA/GYPB/GYPE) (18,22,24). Many other groups have also developed their own targeted NGS panels for genotyping multiple blood group systems (26,37-40).
One of the first uses of targeted NGS genotyping was for antenatal genotyping from cell free fetal DNA initially just for the SNV encoding for the K/k antigen (7) and later expanded to include SNVs encoding for the Fya, Fyb, Jka, Jkb, S, D, c, and E antigens (41). Targeted ABO NGS has also been proven a very powerful tool including the use of long-read sequencing for full cis/trans phasing of ABO alleles and for the resolution of ABO discordances (11,42). In fact, in 2016 Lang et al. (11) was the first to report ABO targeted NGS using automated interpretive software (neXtype) with a concordance of 99.6% (n=415). Lang et al. (11) also demonstrated the scalability of their approach by performing targeted ABO NGS on 1 million stem cell donors. Long-read targeted NGS, which can fully cis/trans-phase regions has also been applied to the Duffy blood group gene ACKR1 (n=81) in order to generate full gene reference haplotype sequences (43). In addition, targeted RHD NGS has been used to define full-length RHD gene sequences for common alleles (44) and to define novel D– variants (45). RHD and RHCE targeted NGS assays have also been designed and tested against a large range of RHD and RHCE alleles. In 2020, Stef et al. (28) published a RHD/RHCE targeted NGS solution with genotyping software that was 99% concordant for a large range of RHD and RHCE alleles including complex SVs (n=278). In addition, several studies have focused on targeted NGS of sickle cell cohorts with particular focus on the genotyping of Rh variants (15,16).
In 2017, Chou et al. (16) was the first to report on WES-based genotyping, but only focused on RHD and RHCE genotyping. In 2017, Schoeman et al. (14,46) was the first to report the use of a clinical exome panel which they used to work up complex serologic cases and to detect novel alleles, including the evaluation of SVs in RHD, RHCE, and GYPC. In 2019, Lane et al. (22) was the first to report on WES-based genotyping of all possible antigens showing that it was possible to correct for WES enrichment biases to call SVs for RHD and RHCE*C/c with 100% concordance (n=75). Schoeman et al. (47) evaluated WES from Indigenous Australians to find several novel blood group alleles, indicating the importance of sequencing a diverse population and that there are likely many blood group alleles yet to be defined.
High-density DNA array genotyping
RBC genotyping using high-density DNA arrays offers an attractive scalability proposition: 1,000s of samples per run, genotyping for almost all genetically known antigens, low cost, high accuracy, and a 4-day turnaround time (Figure 2). DNA arrays have been used to discover the basis of several blood groups including: JR (48), Vel (49), and KANNO (33). However, to date there have only been two publications on the use of high-density DNA arrays for RBC genotyping, both developed through academic and industry collaboration: the REDS-III Transfusion Medicine (TM) Array first reported in 2019 (20) and the Blood transfusion Genomics Consortium (BGC) Universal Donor Typing (UDT) Array first reported in 2020 (26). Both the TM and UDT arrays are based on array technology popularized by large-scale GWAS (29). In addition, these arrays include probes for not only the detection of SNV/indels, but also for CN analysis (Figure 4).
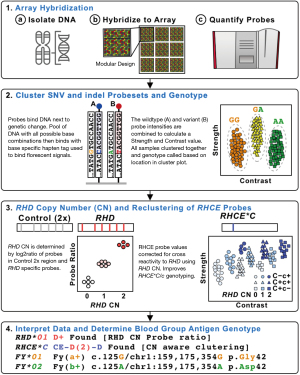
One important aspect of DNA arrays is their flexible nature. Within the space of the array different testing modules can be combined, individual modules customized, and the number of samples increased as the number of different probes decreased. This allows for the creation of an array tailored for each specific use. For example, 800,000 probe arrays can run 96 samples per plate and are ideal for applications like GWAS, whereas 50,000 probe arrays can run 384 per plate and are ideal for donor specific arrays. In addition, one machine can run eight plates per run every 4 days (e.g., so for a 384-sample plate assay ~5,000 samples could be genotyped per machine per week).
The TM Array was tested on ~16,000 samples with ~13,000 United States Blood Donors of mostly European Ancestry and 2,700 Brazillians with sickle cell disease (20). The UDT Array was tested on ~7,000 samples from English and Dutch blood donors mostly of European Ancestry with ongoing studies focused on additional ethnicities (26). The TM Array was compared to known serologic typing for ABO (98.2%, n=12,879) and D (99.6% concordant, n=54) (20). Although the TM Array was not compared with known serologic typing for other antigens, the frequency of many other RBC genetic changes from the array were close to expected population frequencies, including rare genetic changes (<1%) (20). The UDT Array was compared with known serologic/PCR typing for 30 RBC antigens with an overall concordance of 99.91% (A, B, M, N, s, S, D, C, c, E, e, CW, Vw, Lua, Lub, k, K, Kpa, Kpb, Fya, Fyb, Jka, Jkb, Wra, Yta, Coa, Cob, Lan, Vel, n=89,371 comparisons) (26). In addition, the UDT Array was compared with known typing for PLT (99.97% concordance for n=3,106 comparisons) and HLA (99.03% concordance for n=9,289 comparisons) (26). The UDT array was also able to cluster rare genetic RBC antigen changes, which was further improved using the prior knowledge of rare genetic changes in specific samples (26). The application of this prior information will allow for even more accurate clustering of these rare genetic changes in future samples. The UDT array also found several novel ABO type O and MNS alleles from discovery probes added based on data mining of large genomics datasets (26). Of the small number of discordances on the UDT Array, 43% were attributed to errors in the previous typing, most due to serologic typing missing Fyb weak antigens which were detected by the array (26).
It is important to note that for D antigen typing both arrays used a set of CN probes tiled across the RHD gene to determine if RHD and the associated D antigen was present or absent. The TM Array also included a CN probe for the 109 bp indel associated with the C antigen, but did not perform concordance testings (20). The UDT array also included CN probes for the 109 bp indel associated with the C with a 99.9% concordance (n=7,441). It is also important to note that a probes signal intensity can be artificially increased or decreased based on cross binding of probes to homologous genes and if the genes are involved in large deletions (e.g., D– RHD deletions). The UDT Array accounted for these artificial intensity changes clustering the RHD and RHCE probes based on the number of RHD genes present (Figure 4). It is anticipated that similar array-based CN approaches will be successful at detecting other Rh and MNS hybrid genes and studies are ongoing to evaluate for this.
Conclusions
Current serologic and PCR-based genotyping assays have limitations that prevent them from scaling to type all blood donors for all genetically understood antigens (i.e., >330 RBC antigens). NGS allows for an unprecedented evaluation of genetic changes including novel genetic changes and complex SVs and represents the new gold standard for RBC antigen genotyping. High-density DNA arrays allow for low cost evaluation of all known genetic changes including SVs with the ability to run 1,000s of samples at a time. As such, NGS will likely replace conventional PCR genotyping for discordant and complex serologic workups and offer an unprecedented new level of discovery for new RBC antigens. High-density DNA arrays have the potential to allow for routine genotyping of all blood donors for all genetically understood antigens, something that will fundamentally change the practice of transfusion medicine.
Acknowledgments
The author would like to thank Sunitha Vege and Nicholas Gleadall for their helpful input.
Funding: None.
Footnote
Provenance and Peer Review: This article was commissioned by Guest Editor (Yann Fichou) for the series “Molecular Genetics and Genomics of Blood Group Systems” published in Annals of Blood. The article has undergone external peer review.
Conflicts of Interest: The author has completed the ICMJE uniform disclosure form (available at http://dx.doi.org/10.21037/aob-21-8). The series “Molecular Genetics and Genomics of Blood Group Systems” was commissioned by the editorial office without any funding or sponsorship. WJL reports personal consulting fees from CareDx, Inc. and his institution is a founding member of the Blood transfusion Genomics Consortium (BGC) that has received fees from Thermo Fisher Scientific Inc. to help co-develop a high-density DNA genotyping array.
Ethical Statement: The author is accountable for all aspects of the work, ensuring that questions related to the accuracy or integrity of any part of the work are appropriately investigated and resolved.
Open Access Statement: This is an Open Access article distributed in accordance with the Creative Commons Attribution-NonCommercial-NoDerivs 4.0 International License (CC BY-NC-ND 4.0), which permits the non-commercial replication and distribution of the article with the strict proviso that no changes or edits are made and the original work is properly cited (including links to both the formal publication through the relevant DOI and the license). See: https://creativecommons.org/licenses/by-nc-nd/4.0/.
References
- Chou ST, Jackson T, Vege S, et al. High prevalence of red blood cell alloimmunization in sickle cell disease despite transfusion from Rh-matched minority donors. Blood 2013;122:1062-71. [Crossref] [PubMed]
- Hendrickson JE, Tormey CA, Shaz BH. Red blood cell alloimmunization mitigation strategies. Transfus Med Rev 2014;28:137-44. [Crossref] [PubMed]
- Tormey CA, Hendrickson JE. Transfusion-related red blood cell alloantibodies: induction and consequences. Blood 2019;133:1821-30. [Crossref] [PubMed]
- Schonewille H, van de Watering LMG, Brand A. Additional red blood cell alloantibodies after blood transfusions in a nonhematologic alloimmunized patient cohort: is it time to take precautionary measures? Transfusion 2006;46:630-5. [Crossref] [PubMed]
- Westhoff CM. Blood group genotyping. Blood 2019;133:1814-20. [Crossref] [PubMed]
- Stabentheiner S, Danzer M, Niklas N, et al. Overcoming methodical limits of standard RHD genotyping by next-generation sequencing. Vox Sang 2011;100:381-8. [Crossref] [PubMed]
- Rieneck K, Bak M, Jønson L, et al. Next-generation sequencing: proof of concept for antenatal prediction of the fetal Kell blood group phenotype from cell-free fetal DNA in maternal plasma. Transfusion 2013;53:2892-8. [Crossref] [PubMed]
- Fichou Y, Audrézet MP, Guéguen P, et al. Next-generation sequencing is a credible strategy for blood group genotyping. Br J Haematol 2014;167:554-62. [Crossref] [PubMed]
- Giollo M, Minervini G, Scalzotto M, et al. BOOGIE: Predicting blood groups from high throughput sequencing data. PLoS One 2015;10:e0124579 [Crossref] [PubMed]
- Lane WJ, Westhoff CM, Uy JM, et al. Comprehensive red blood cell and platelet antigen prediction from whole genome sequencing: proof of principle. Transfusion 2016;56:743-54. [Crossref] [PubMed]
- Lang K, Wagner I, Schöne B, et al. ABO allele-level frequency estimation based on population-scale genotyping by next generation sequencing. BMC Genomics 2016;17:374. [Crossref] [PubMed]
- Baronas J, Westhoff CM, Vege S, et al. RHD zygosity determination from whole genome sequencing data. J Blood Disord Transfus 2016;7:365. [Crossref]
- Möller M, Jöud M, Storry JR, et al. Erythrogene: a database for in-depth analysis of the extensive variation in 36 blood group systems in the 1000 Genomes Project. Blood Adv 2016;1:240-9. [Crossref] [PubMed]
- Schoeman EM, Lopez GH, McGowan EC, et al. Evaluation of targeted exome sequencing for 28 protein-based blood group systems, including the homologous gene systems, for blood group genotyping. Transfusion 2017;57:1078-88. [Crossref] [PubMed]
- Dezan MR, Ribeiro IH, Oliveira VB, et al. RHD and RHCE genotyping by next-generation sequencing is an effective strategy to identify molecular variants within sickle cell disease patients. Blood Cells Mol Dis 2017;65:8-15. [Crossref] [PubMed]
- Chou ST, Flanagan JM, Vege S, et al. Whole-exome sequencing for RH genotyping and alloimmunization risk in children with sickle cell anemia. Blood Adv 2017;1:1414-22. [Crossref] [PubMed]
- Möller M, Hellberg Å, Olsson ML. Thorough analysis of unorthodox ABO deletions called by the 1000 Genomes project. Vox Sang 2018;113:185-97. [Crossref] [PubMed]
- Lane WJ, Westhoff CM, Gleadall NS, et al. Automated typing of red blood cell and platelet antigens: a whole-genome sequencing study. Lancet Haematol 2018;5:e241-51. [Crossref] [PubMed]
- Montemayor-Garcia C, Karagianni P, Stiles DA, et al. Genomic coordinates and continental distribution of 120 blood group variants reported by the 1000 Genomes Project. Transfusion 2018;58:2693-704. [Crossref] [PubMed]
- Guo Y, Busch MP, Seielstad M, et al. Development and evaluation of a transfusion medicine genome wide genotyping array. Transfusion 2019;59:101-11. [Crossref] [PubMed]
- Wheeler MM, Lannert KW, Huston H, et al. Genomic characterization of the RH locus detects complex and novel structural variation in multi-ethnic cohorts. Genet Med 2019;21:477-86. [Crossref] [PubMed]
- Lane WJ, Vege S, Mah HH, et al. Automated typing of red blood cell and platelet antigens from whole exome sequences. Transfusion 2019;59:3253-63. [Crossref] [PubMed]
- Lane WJ, Gleadall NS, Aeschlimann J, et al. Multiple GYPB gene deletions associated with the U- phenotype in those of African ancestry. Transfusion 2020;60:1294-307. [Crossref] [PubMed]
- Halls JBL, Vege S, Simmons DP, et al. Overcoming the challenges of interpreting complex and uncommon RH alleles from whole genomes. Vox Sang 2020;115:790-801. [Crossref] [PubMed]
- Gassner C, Denomme GA, Portmann C, et al. Two Prevalent ∼100-kb GYPB Deletions Causative of the GPB-Deficient Blood Group MNS Phenotype S-s-U- in Black Africans. Transfus Med Hemother 2020;47:326-36. [Crossref] [PubMed]
- Gleadall NS, Veldhuisen B, Gollub J, et al. Development and validation of a universal blood donor genotyping platform: a multinational prospective study. Blood Adv 2020;4:3495-506. [Crossref] [PubMed]
- Chang TC, Haupfear KM, Yu J, et al. A novel algorithm comprehensively characterizes human RH genes using whole-genome sequencing data. Blood Adv 2020;4:4347-57. [Crossref] [PubMed]
- Stef M, Fennell K, Apraiz I, et al. RH genotyping by nonspecific quantitative next-generation sequencing. Transfusion 2020;60:2691-701. [Crossref] [PubMed]
- Sudlow C, Gallacher J, Allen N, et al. UK biobank: an open access resource for identifying the causes of a wide range of complex diseases of middle and old age. PLoS Med 2015;12:e1001779 [Crossref] [PubMed]
- Lane WJ, Aguad M, Smeland-Wagman R, et al. A whole genome approach for discovering the genetic basis of blood group antigens: independent confirmation for P1 and Xga. Transfusion 2019;59:908-15. [Crossref] [PubMed]
- Leffler EM, Band G, Busby GBJ, et al. Resistance to malaria through structural variation of red blood cell invasion receptors. Science 2017;356:eaam6393 [Crossref] [PubMed]
- Cvejic A, Haer-Wigman L, Stephens JC, et al. SMIM1 underlies the Vel blood group and influences red blood cell traits. Nat Genet 2013;45:542-5. [Crossref] [PubMed]
- Omae Y, Ito S, Takeuchi M, et al. Integrative genome analysis identified the KANNO blood group antigen as prion protein. Transfusion 2019;59:2429-35. [Crossref] [PubMed]
- Vrignaud C, Mikdar M, Koehl B, et al. Alloantibodies directed to the SLC44A2/CTL2 transporter define two new red cell antigens and a novel human blood group system. In: 2019 Annual Meeting. AABB, 2019.
- Azouzi S, Mikdar M, Hermand P, et al. Lack of the multidrug transporter MRP4/ABCC4 defines the PEL-negative blood group and impairs platelet aggregation. Blood 2020;135:441-8. [Crossref] [PubMed]
- Thornton N, Karamatic Crew V, Tilley L, et al. Disruption of the tumour-associated EMP3 enhances erythroid proliferation and causes the MAM-negative phenotype. Nat Commun 2020;11:3569. [Crossref] [PubMed]
- Wieckhusen C, Bugert P. 454-SequencingTM for the KEL, JR, and LAN Blood Groups. Methods Mol Biol 2015;1310:123-33. [Crossref] [PubMed]
- Jakobsen MA, Dellgren C, Sheppard C, et al. The use of next-generation sequencing for the determination of rare blood group genotypes. Transfus Med 2019;29:162-8. [Crossref] [PubMed]
- Orzińska A, Guz K, Mikula M, et al. A preliminary evaluation of next-generation sequencing as a screening tool for targeted genotyping of erythrocyte and platelet antigens in blood donors. Blood Transfus 2018;16:285-92. [PubMed]
- Boccoz SA, Fouret J, Roche M, et al. Massively parallel and multiplex blood group genotyping using next-generation-sequencing. Clin Biochem 2018;60:71-6. [Crossref] [PubMed]
- Orzińska A, Guz K, Mikula M, et al. Prediction of fetal blood group and platelet antigens from maternal plasma using next-generation sequencing. Transfusion 2019;59:1102-7. [Crossref] [PubMed]
- Wu PC, Lin YH, Tsai LF, et al. ABO genotyping with next-generation sequencing to resolve heterogeneity in donors with serology discrepancies. Transfusion 2018;58:2232-42. [Crossref] [PubMed]
- Fichou Y, Berlivet I, Richard G, et al. Defining blood group gene reference alleles by long-read sequencing: proof of concept in the ACKR1 gene encoding the duffy antigens. Transfus Med Hemother 2020;47:23-32. [Crossref] [PubMed]
- Tounsi WA, Madgett TE, Avent ND. Complete RHD next-generation sequencing: establishment of reference RHD alleles. Blood Adv 2018;2:2713-23. [Crossref] [PubMed]
- Tammi SM, Tounsi WA, Sainio S, et al. Next-generation sequencing of 35 RHD variants in 16 253 serologically D- pregnant women in the Finnish population. Blood Adv 2020;4:4994-5001. [Crossref] [PubMed]
- Schoeman EM, Roulis EV, Liew YW, et al. Targeted exome sequencing defines novel and rare variants in complex blood group serology cases for a red blood cell reference laboratory setting. Transfusion 2018;58:284-93. [Crossref] [PubMed]
- Schoeman EM, Roulis EV, Perry MA, et al. Comprehensive blood group antigen profile predictions for Western Desert Indigenous Australians from whole exome sequence data. Transfusion 2019;59:768-78. [Crossref] [PubMed]
- Zelinski T, Coghlan G, Liu XQ, et al. ABCG2 null alleles define the Jr(a-) blood group phenotype. Nat Genet 2012;44:131-2. [Crossref] [PubMed]
- Storry JR, Jöud M, Christophersen MK, et al. Homozygosity for a null allele of SMIM1 defines the Vel-negative blood group phenotype. Nat Genet 2013;45:537-41. [Crossref] [PubMed]
Cite this article as: Lane WJ. Recent advances in blood group genotyping. Ann Blood 2021;6:31.